- 🔍 Overview
- Abstract
- Introduction
- 📉 Liquidity Provider Rewards Program
- Q-Score
- Analysis of Q-Score Historical Performance
- Preliminary Evaluation of G Score & Capping based on Historical Data
- G-Score Analysis
- Reward Capping Analysis
- Wintermute Proposal - Analysis of Historical Data
- Initial Recommendations
- Research and Evaluation - Utilizing Synthetic Data Sets
- Simulations Result Summary
- About the dYdX Grant Program
🔍 Overview
Chaos Labs, a passionate participant in the decentralized finance (DeFi) community, is proud to collaborate with prominent DeFi protocols. Among these, dYdX stands out as a leading decentralized exchange that facilitates perpetuals and holds a position of prominence within the industry. In line with its dedication and objective of promoting DeFi adoption, Chaos Labs endeavors to construct and disseminate open-source resources that benefit the wider developer community. In furtherance of this effort, Chaos Labs has published the inaugural report on Market Maker Liquidity Rewards Distribution.
Abstract
This paper presents an in-depth analysis of the dYdX protocol's market maker liquidity rewards optimization. By examining the different factors that contribute to the rewards offered by the dYdX protocol, we aim to identify the optimal strategies to maximize returns for market makers contributing impactful volume. Our findings provide essential insights for market makers, liquidity providers, and traders looking to maximize their returns in the dYdX ecosystem.
Introduction
Decentralized finance (DeFi) has experienced tremendous growth in recent years, with the dYdX protocol emerging as a prominent player in the decentralized exchange landscape. The protocol offers various financial services, such as margin trading, derivatives, and perpetuals, through a decentralized, non-custodial platform. This research investigates the liquidity rewards optimization in the dYdX market maker program, focusing on the factors that impact these rewards and the strategies that can be employed to maximize returns for market makers.
📉 Liquidity Provider Rewards Program
The goal of the Liquidity Provider Rewards program is to incentivize market makers to provide meaningful liquidity. Ideally, incentives should motivate makers to tighten spreads and compete on volume. However, after several epochs, we’ve identified cases in which incentive distribution could be improved to better align with impactful liquidity provisioning. This summary will review the current formula, examine live proposals and elaborate on our analysis highlighting the tradeoffs each approach introduces.
Q-Score
To encourage the provision of market liquidity, dYdX will distribute $DYDX tokens to liquidity providers based on a formula that rewards their engagement in the markets. The formula takes into account three parameters:
- two-sided depth
- spread (compared to the mid-market price)
- uptime on dYdX's Layer 2 Protocol
Any Ethereum address can participate in this incentive program, provided that they meet the minimum maker volume threshold of 0.25% of the total maker volume during the preceding epoch. The distribution of $DYDX tokens will occur on a 28-day epoch basis over a period of five years and will not be subject to any vesting or lockups. Each epoch will see the distribution of 1,150,685 $DYDX tokens.
The distribution of $DYDX tokens to each liquidity provider per epoch is determined by the following function. The amount of $DYDX tokens earned depends on the proportionate share of each participant's Q-Final.

Although the formula used for computing rewards based on two-sided depth, spread, and uptime is effective and promotes crucial factors, it falls short in ensuring an equitable distribution of incentives. This is because it does not consider the maker volume. Below are some examples that illustrate cases where rewards distribution is disproportionate as a result of maker volume not being factored in.
Analysis of Q-Score Historical Performance
The LP reward distribution has been computed using the Q-Score formula over multiple epochs. Upon reviewing the rewards distribution across several epochs, it has become evident that the Q-Score formula is not optimal and can result in an unfair distribution of rewards.
Figure 1.1 clearly highlights some of the challenges. Let’s look at the maker depicted as account #5 (X-axis). This maker has contributed relatively low volume and has a disproportionate Q-score.
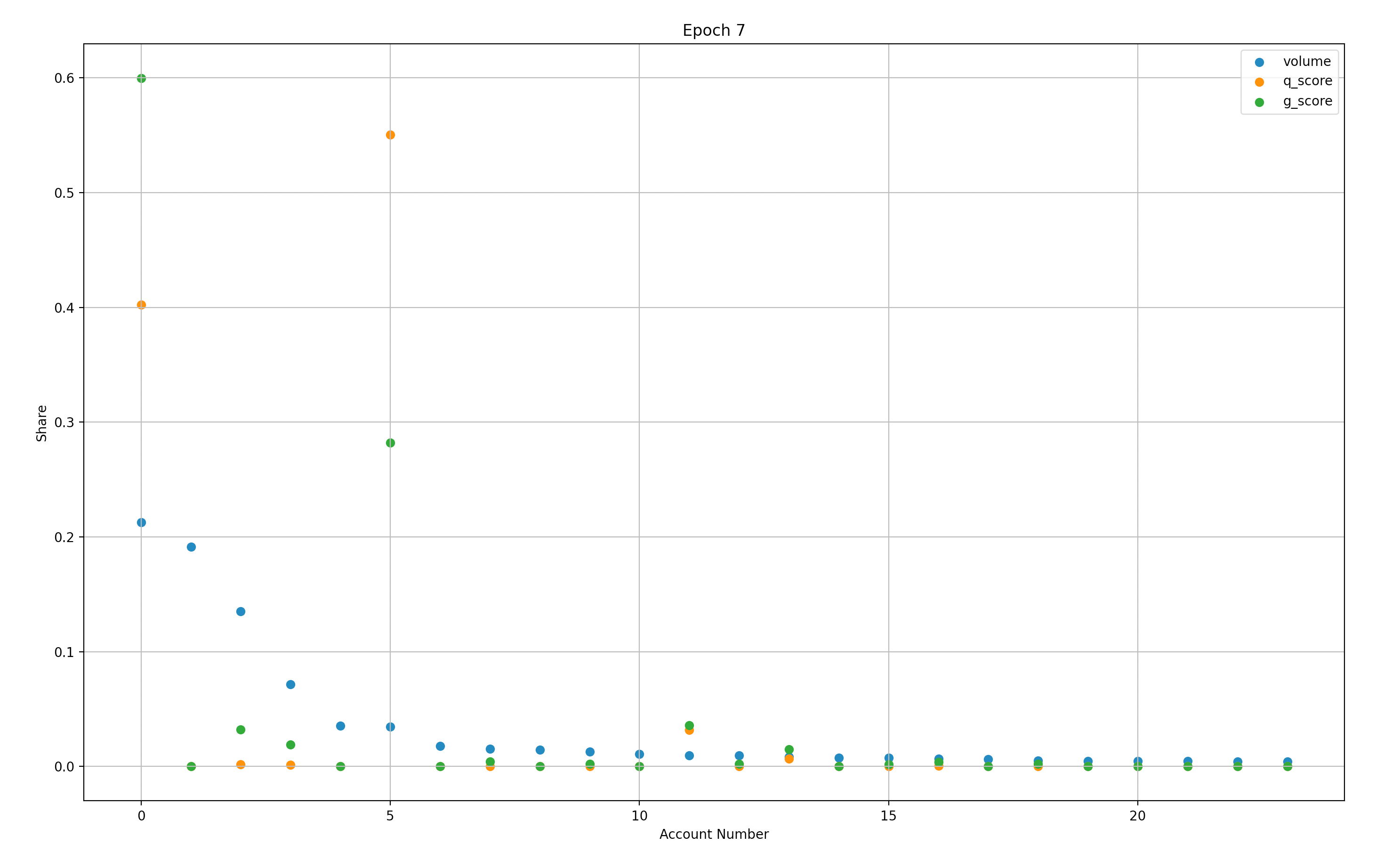
Figure 1.2: This image is a screenshot of distributions observed in Epoch 7. Here we can clearly view a low relative volume contribution with an ~18x multiplier on Q-Score.

Furthermore, Account #11 has a similar Q-score/volume inversion, albeit at a smaller scale. A relatively quick analysis of historical distributions highlights suboptimal outcomes.
Preliminary Evaluation of G Score & Capping based on Historical Data
Our objective was to assess the effectiveness of three proposed modifications to the existing Q-score based reward distribution formula. These modifications are as follows:
G-Score
This modification involves computing a new score, called the G-Score, which is derived from the equal-weighted geometric average of a given address's Q-score and volume share. The rewards are then distributed in proportion to each participant's G-score. 2/3 x Capping
The second modification entails capping the maximum possible reward at 2-3 times the relative volume contributed by a single address. Any remaining rewards are then redistributed pro-rata according to the volume share of the remaining participants.
Wintermute New Rewards Distribution The third modification proposes a new rewards distribution formula that incorporates maker volume and capped rewards, reduces the weight of depth, spread, and stkDYDX, and provides optional capping. Our evaluation aimed to determine the effectiveness of these modifications in addressing the issues of unfair rewards distribution that arise from the Q-score based formula.
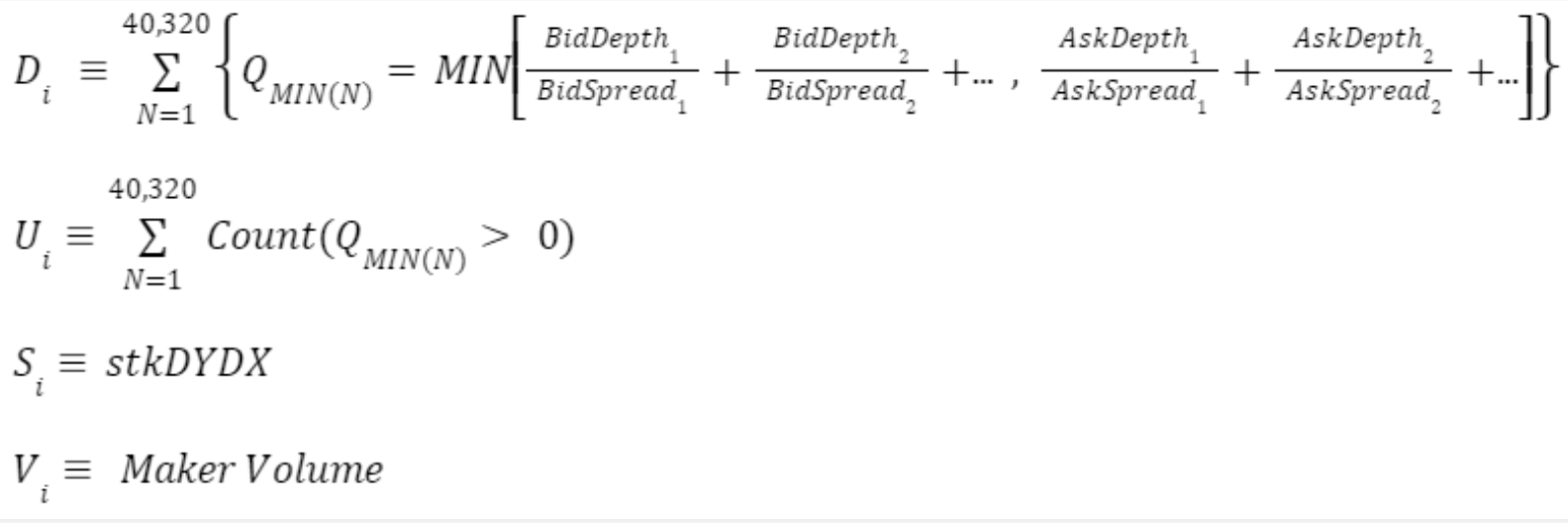
The total rewards score for an individual LP, $Q_i$, is then calculated as follows:
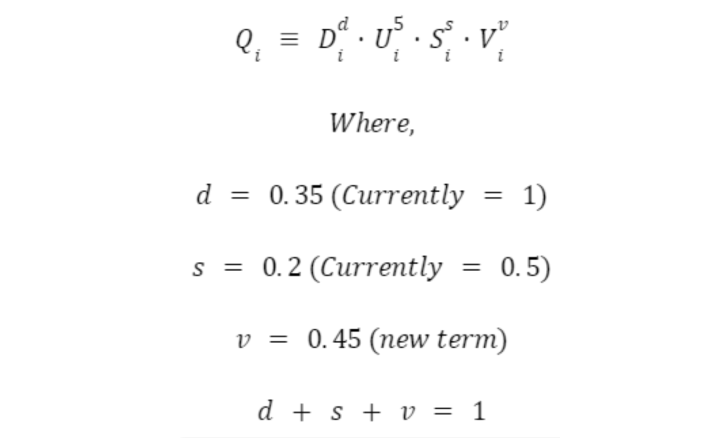
G-Score Analysis
Based on our analysis of historical distributions, it appears that the proposed modifications result in a fairer rewards distribution from a volume perspective when compared to the current Q-score based formula. However, we have identified some potential risks and pitfalls that should be taken into consideration:
1. Over-compensation Risk
One potential issue with the G-score modification is that it compounds the volume portion with the Q-score, resulting in a G-score that can be several times greater than the Q-score. As a result, there are cases where a single significant Liquidity Provider (LP) can receive approximately 60% of the reward pool, and in some cases, a single maker may receive up to 70% of the reward distributions. This level of lopsided reward distribution, while it may be legitimate in some cases, can lead to a single LP dominating the market. This can discourage smaller makers and create a monopolized protocol liquidity, ultimately leading to a decrease in overall decentralization. In the long term, a lack of maker competition and diversity may harm the protocol.

2. Fairness Inefficiency
In our effort to optimize rewards distribution fairness while considering trading volume, we have observed instances where the G-score modification underperforms. There are concrete examples of Liquidity Providers (LPs) with a G-score that is approximately 30 times their trading volume. This suggests that in some cases, the G-score may not accurately reflect a participant's contribution to the protocol's liquidity.
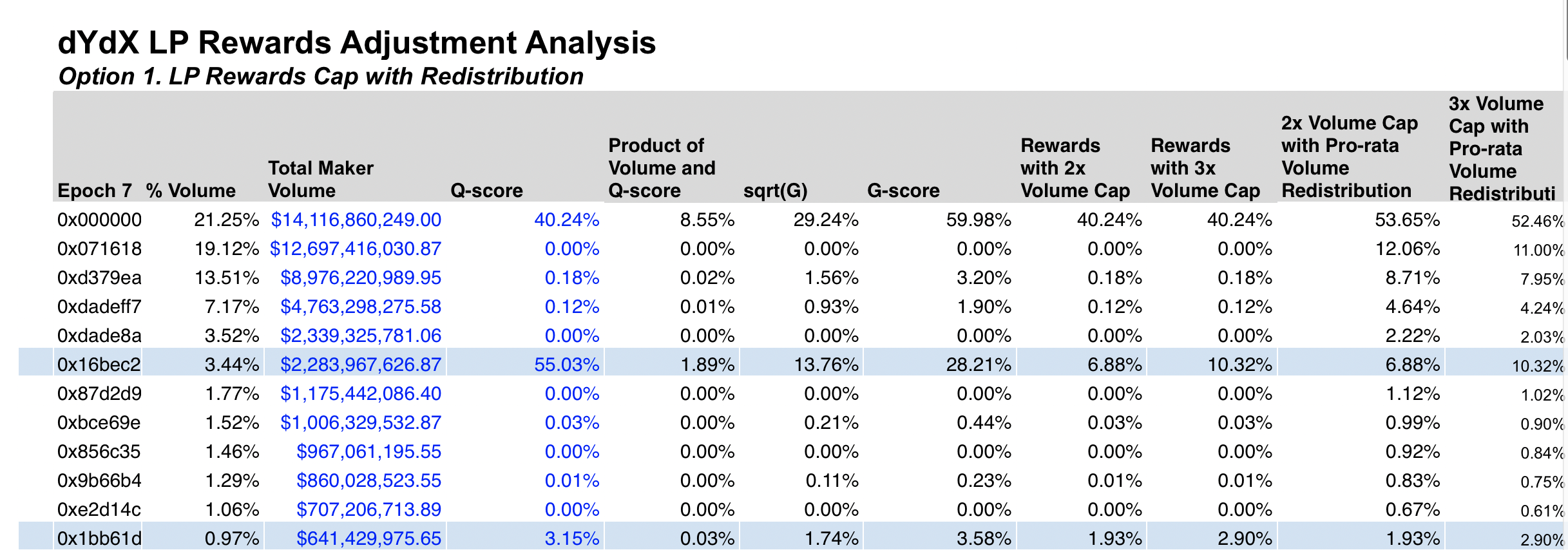
3. Zero-Sum Reward Distribution
It is essential to consider the inverse risk that may arise from "Fairness Inefficiency." Although we strive to achieve a fair rewards distribution, it is important to remember that rewards distribution is a zero-sum game. When larger makers skew the distribution in their favor, it can negatively impact honest makers. This may lead some makers to consider providing liquidity on different platforms or protocols, ultimately harming the protocol's overall liquidity and decreasing decentralization. Therefore, it is crucial to strike a balance between fairness and efficiency when designing a rewards distribution mechanism.
Reward Capping Analysis
Capping introduces a much stronger correlation to trading volume compared to Q-score and G-score. However, after analyzing historical distributions, we’ve identified new pitfalls and types of unfairness.
1. Capping heavily incentivizes trading volume even with very low Q scores. This enables rewards of up to 100-250x the Q score.

2. Capping can significantly reduce Q-Score rewards. This can minimize the weight of the Q-score relative to trading volume.

Wintermute Proposal - Analysis of Historical Data
Proposed Weighting
Figure 2.1
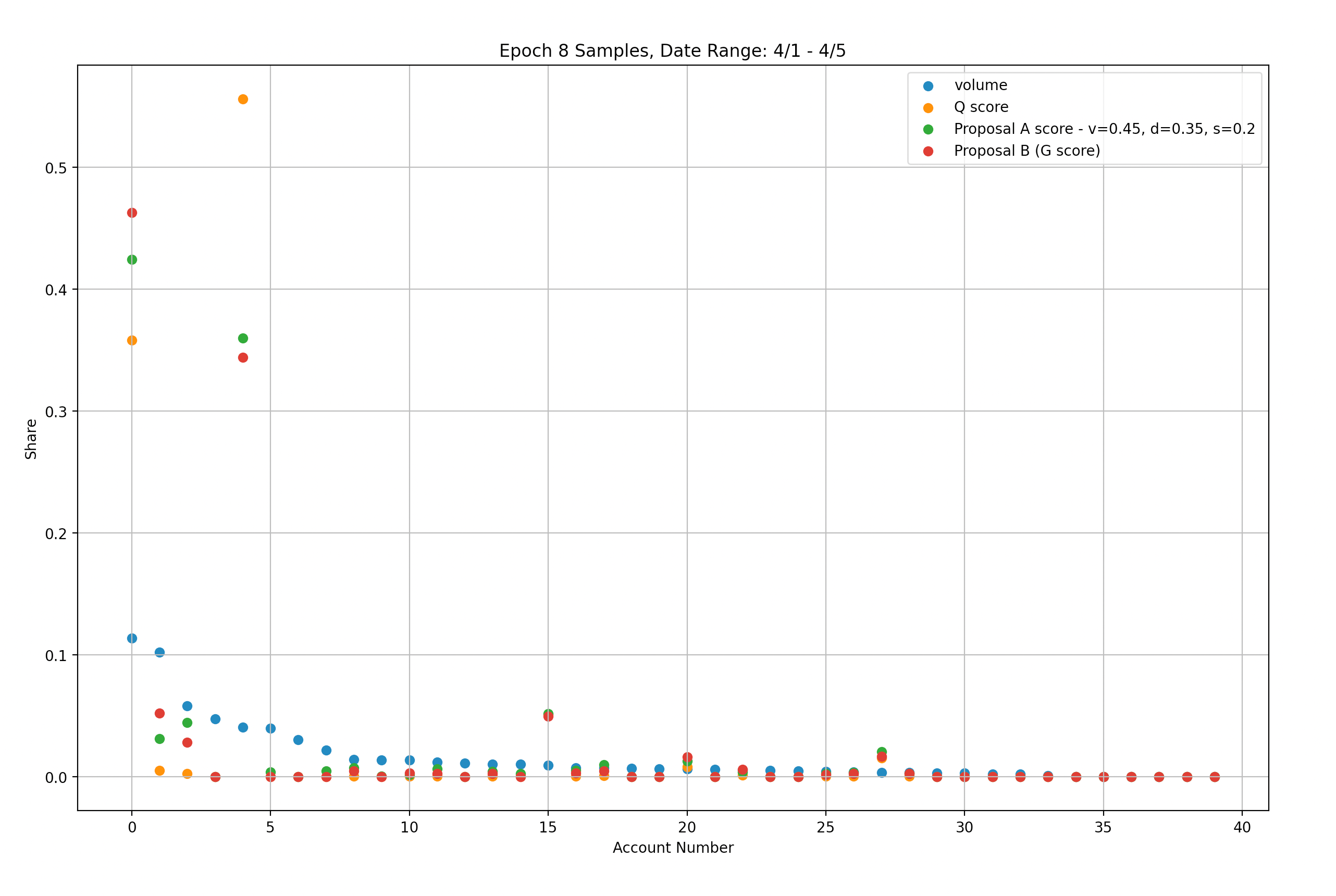
Our analysis indicates that similar results can be achieved using the Weighted-Score modification with suggested scaling parameters (v=0.45, d=0.35, s=0.2) and the uncapped G-score modification (represented by green and red markers respectively). Specifically, we found that with the Weighted-Score modification, 78% of rewards were still distributed to the top two accounts, while the uncapped G-score modification resulted in 80.5% of rewards going to the same two accounts.
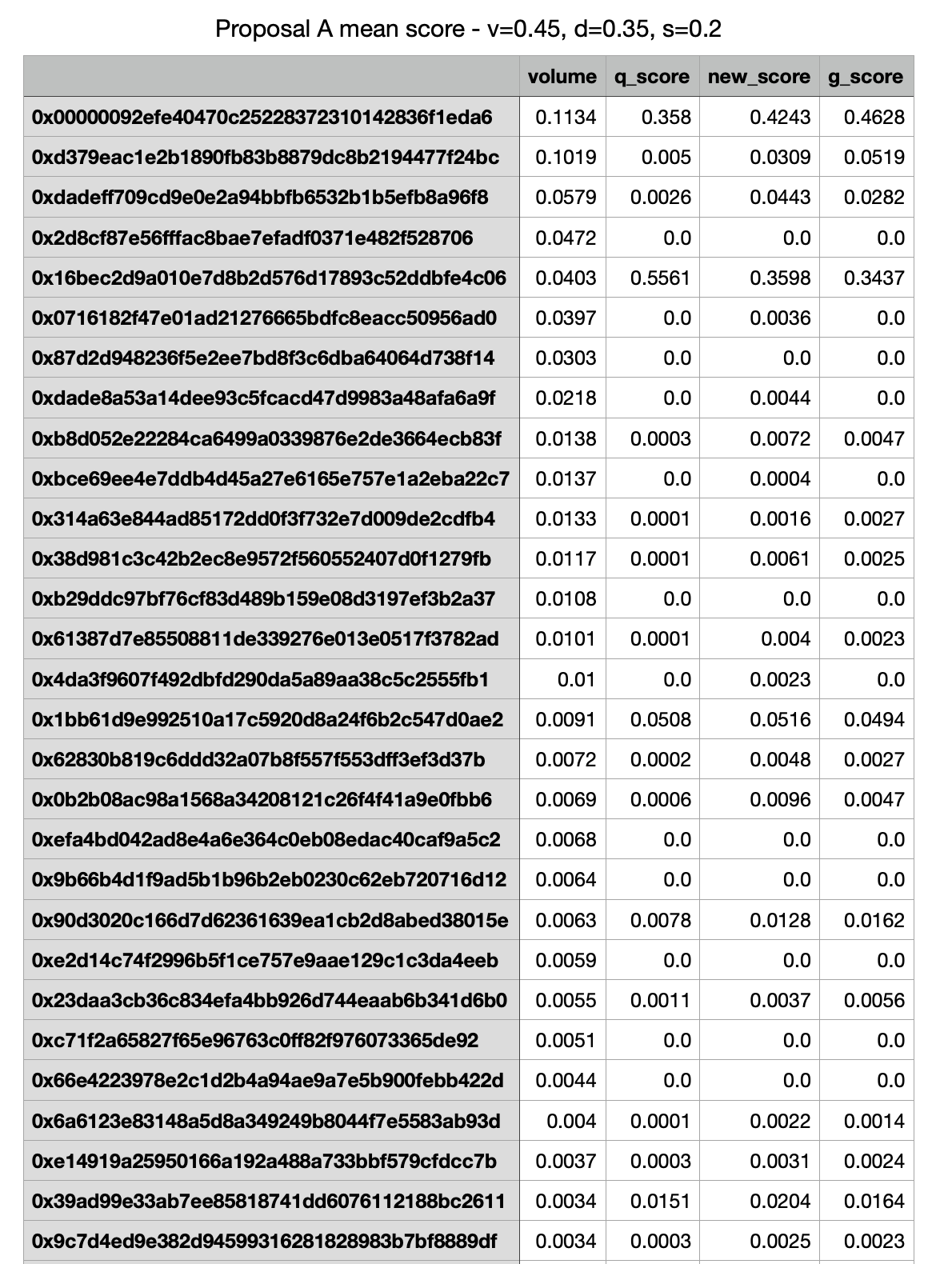
Heavily Favor Maker Volume:
Figure 2.2
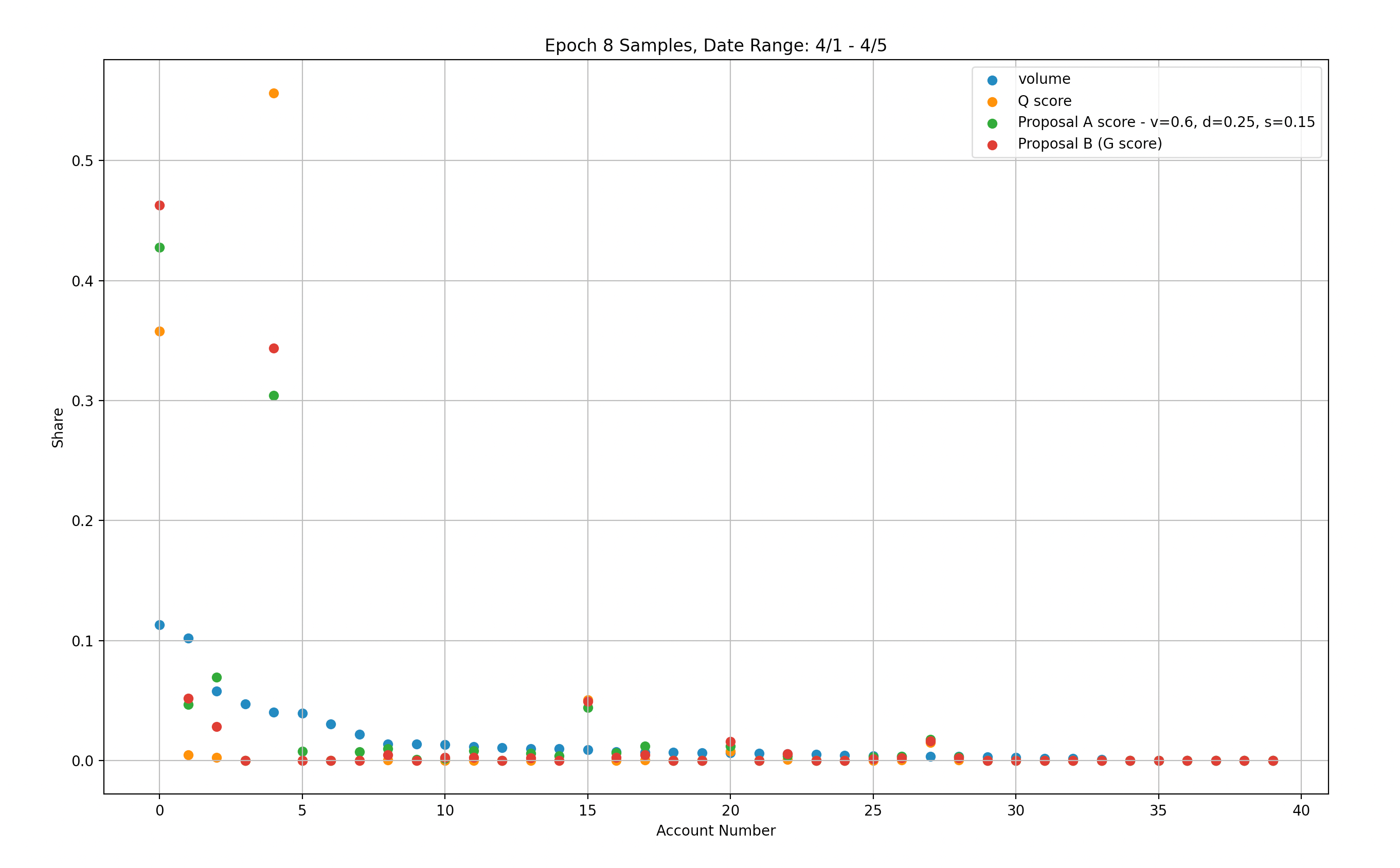
We have conducted an analysis using a weighted score modification, setting scaling parameters to heavily favor volume (v=0.6, d=0.25, s=0.15). This modification leads to better penalization of account #4 while providing similar rewards to account #0 compared to the original set of parameters. However, even with this modification, 73% of rewards are still distributed to the top two accounts, indicating that further adjustments may be necessary to achieve a more equitable rewards distribution.
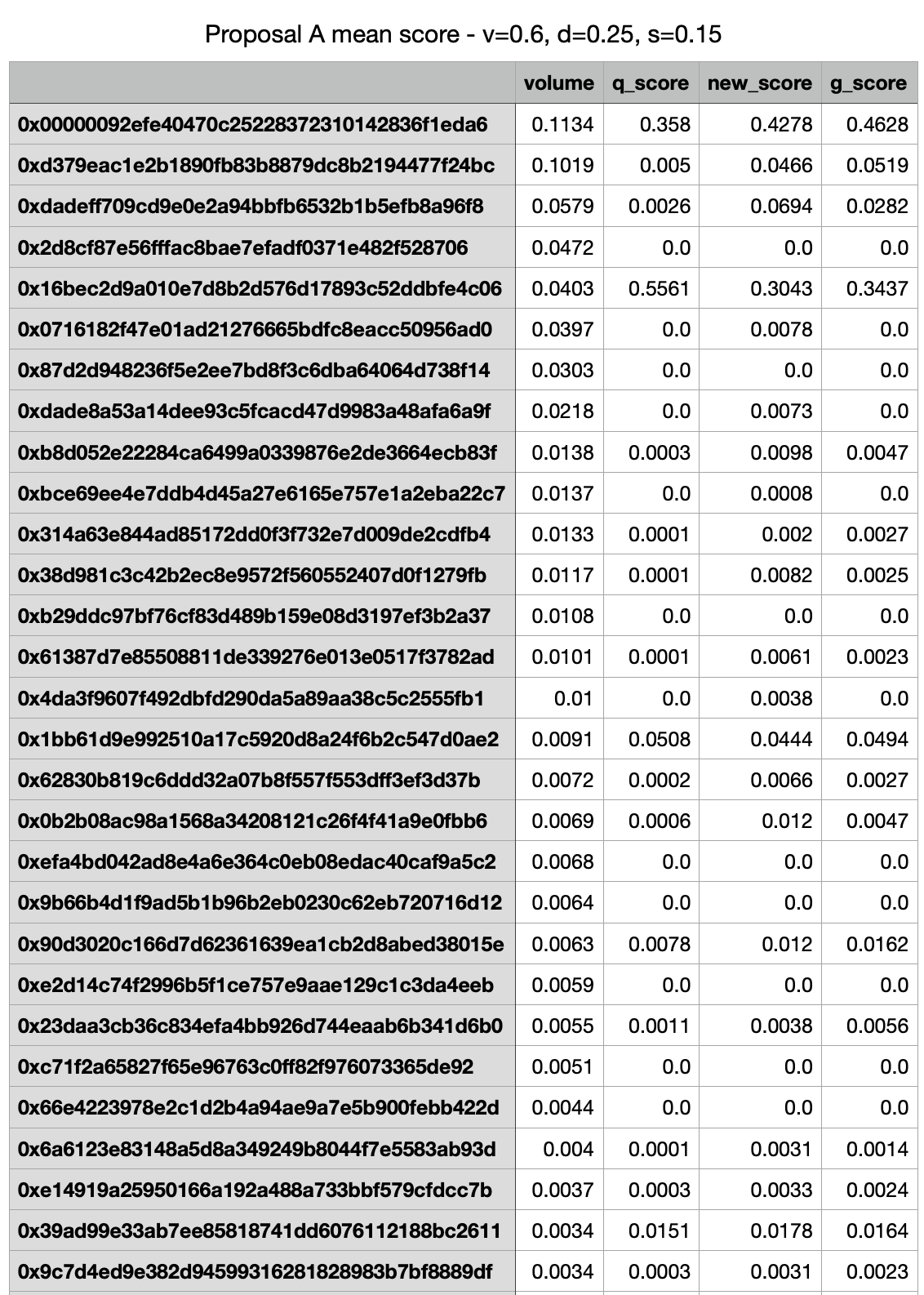
Heavily Favor Depth / Spread
Figure 2.3
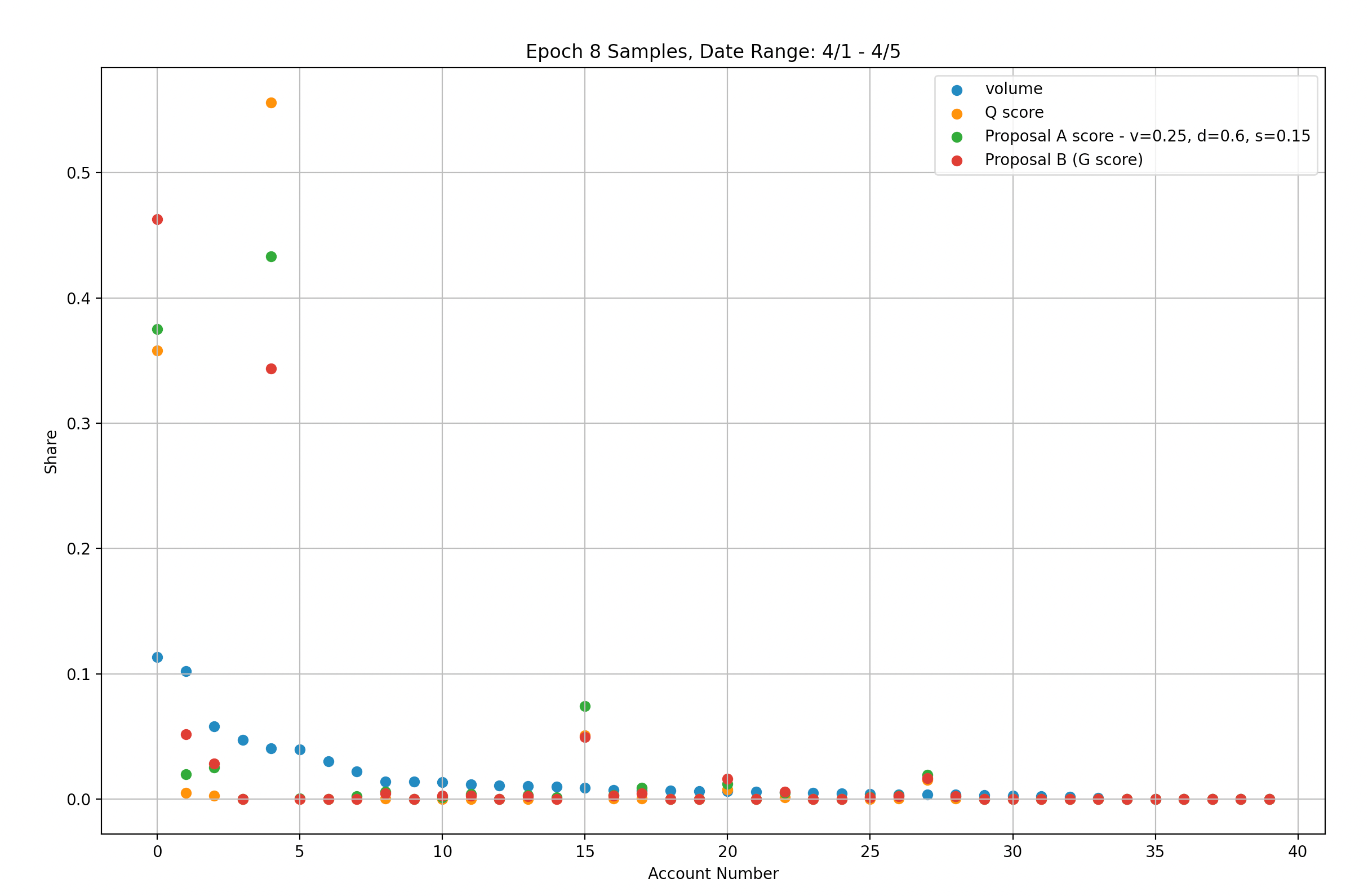
In our analysis using the Weighted-Score modification, we set the scaling parameters to heavily favor Depth/Spread (v=0.25, d=0.6, s=0.15). However, this modification resulted in worse penalization of account #4, while account #0 received lower rewards compared to the original set of parameters. Furthermore, 81% of rewards are still distributed to the top two accounts, indicating that this modification does not improve the fairness of rewards distribution.
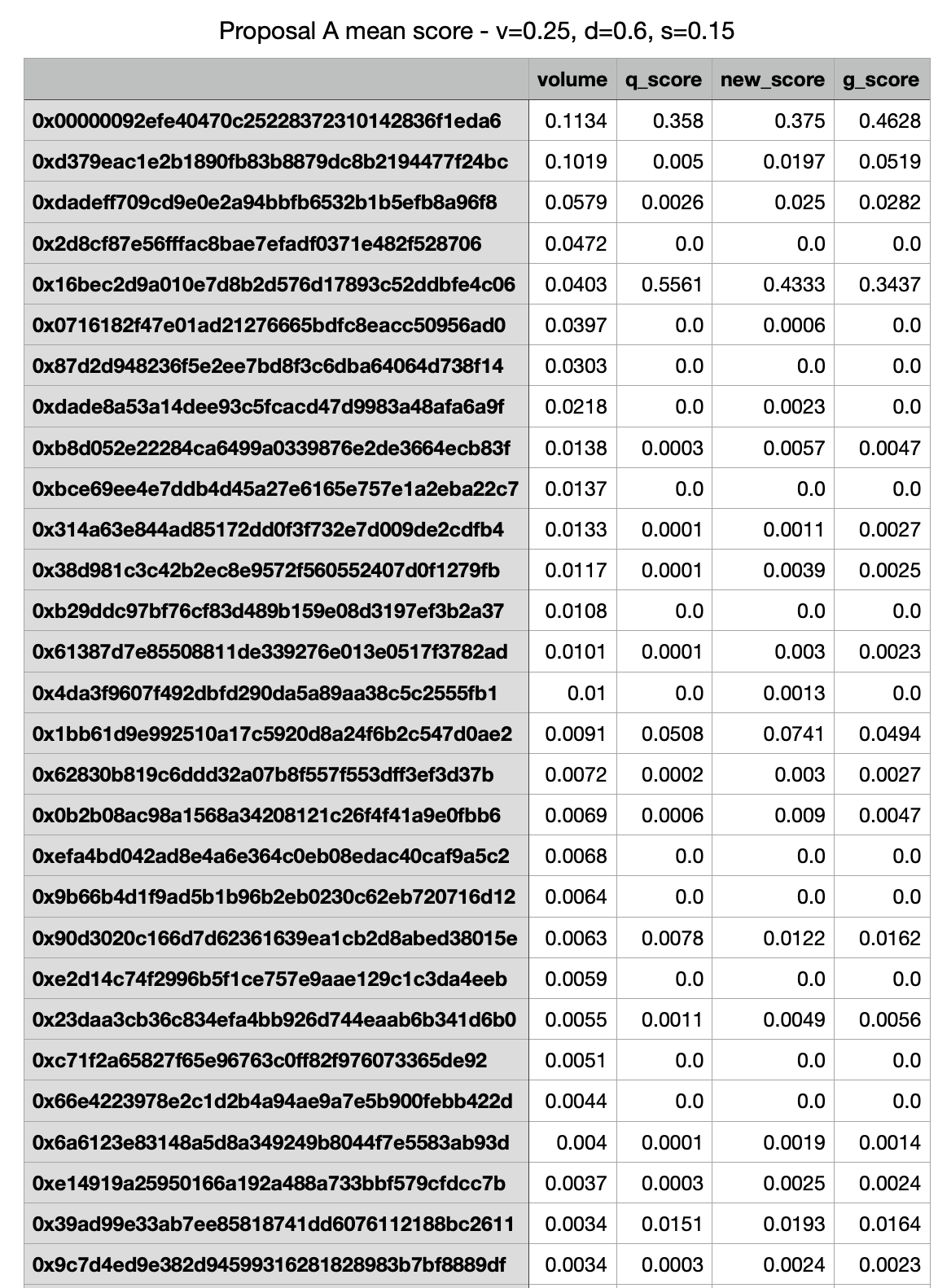
Initial Recommendations
Considering dYdX's intention to promptly address the current state of rewards distribution, we aim to provide short-term recommendations based solely on the current historical data available. However, we recognize that these recommendations may not provide a long-term solution, and therefore, we intend to conduct thorough research and analysis-based simulations to develop a more balanced and fairer solution for the future.
Immediate Recs
Based on our immediate analysis of the two improvement proposals, namely G-score and Weighted-Score (with or without capping), we conclude that both modifications can address the issue of rewards distribution unfairness. G-score can be viewed as a special case of the Weighted-Score, which gives equal weight to volume and Q-scores. However, the Weighted-Score modification has the advantage of allowing the weights to be adjusted.
While it is not entirely clear from the historical data which weights are best for achieving desired liquidity, simulations using a wider range of input synthetic data indicate that the suggested weights (v=0.45, d=0.35, s=0.2) for the Weighted-Score modification are better in terms of rewarding smaller LPs while avoiding over-penalizing large "passive" LPs (i.e., LPs with high trading volume but low Q-score).
On the other hand, capping provides greater fairness, but it also poses a higher risk of manipulation. Therefore, we recommend implementing the Weighted-Score/G-score modification over capping in the short term, with a period of review to further evaluate the effectiveness of the modification.
Longer-term Recommendations
Given the challenges we faced in identifying immediate pitfalls with the proposed modifications and the historical cases of inefficiencies or adversarial outcomes, we strongly recommend conducting in-depth research and analysis to develop a more robust and battle-tested formula to achieve dYdX's objective of fairness across its four pillars: depth, spread, uptime, and volume.
While the Weighted-Score/G-score modifications represent progress towards achieving this objective, we have determined that the distribution of weights between the pillars is still suboptimal. Therefore, further research is necessary to develop a more effective and equitable rewards distribution mechanism.
Research and Evaluation - Utilizing Synthetic Data Sets
As experts in building agent-based simulations that model real user behavior, we at Chaos Labs have leveraged our simulation platform to simulate possible future epochs. To construct these simulations, we developed the following user modes:
- Large Market Makers - LPs with significant liquidity that can compete for high Q-scores.
- Small Market Makers - LPs that have a low probability of achieving high Q-scores.
- Adversarial Market Makers - LPs that actively try and often succeed at gaming the Q-scores and other aspects. Based on historical data, we evaluated the possibility of an LP falling into one or more of these categories to create simulated epochs that closely resemble reality and are statistically feasible.
Based on historical data, we evaluated the possibility of an LP falling into one or more of these categories to create simulated epochs that closely resemble reality and are statistically feasible.
Using these models, we generated 10,000 possible epochs and used them to compare the efficiency of the G-score formula on key metrics.
Note - There is a lot more information and insights to derive from these simulations. This would require building more sophisticated models and adjusting our predictions and conclusions accordingly. The research presented factors in the short time frame and the immediate goal of going live with G-Score in the short term.
Simulations Analysis Highlights
Q-score, G-score, Weighted-Score on top performers
The following graphs aggregate results from 10,000 simulations to compare G-score versus Q-score in terms of rewards distributed to the top performer in each epoch:
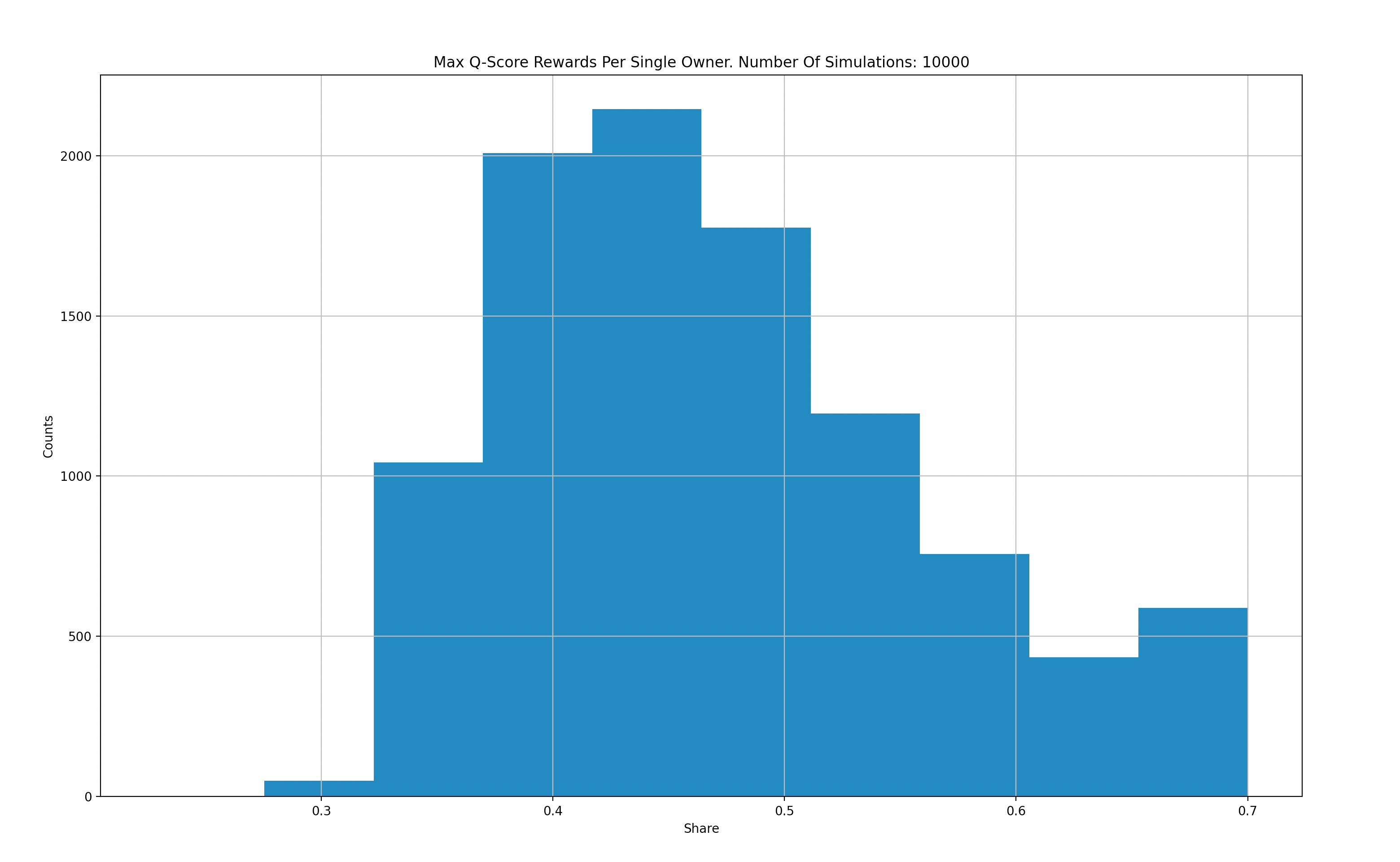
X-Axis: Max Q Score for a single maker.
Y-Axis: Count per 10k simulations.
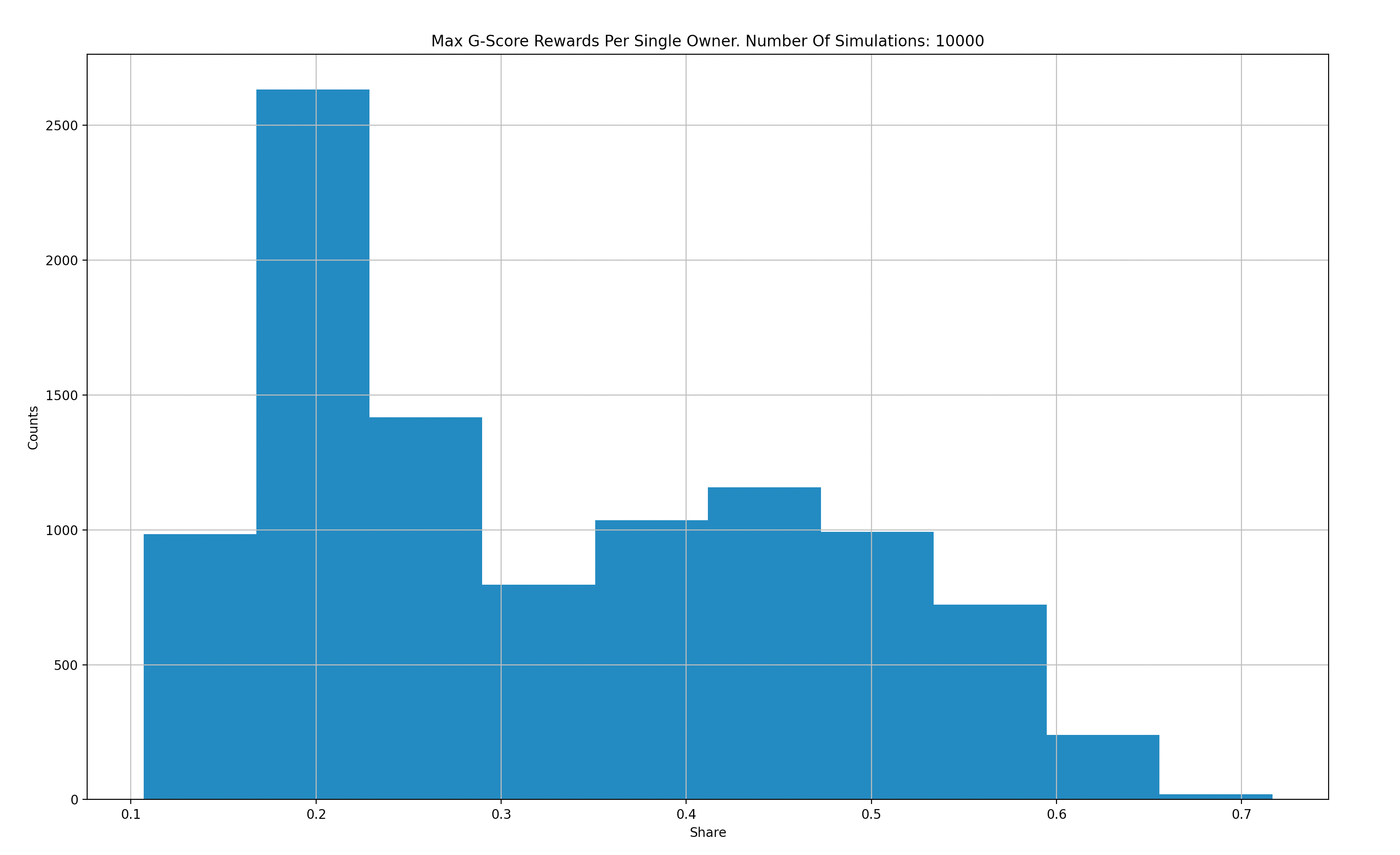
X-Axis: Max G Score for a single maker.
Y-Axis: Count per 10k simulations.
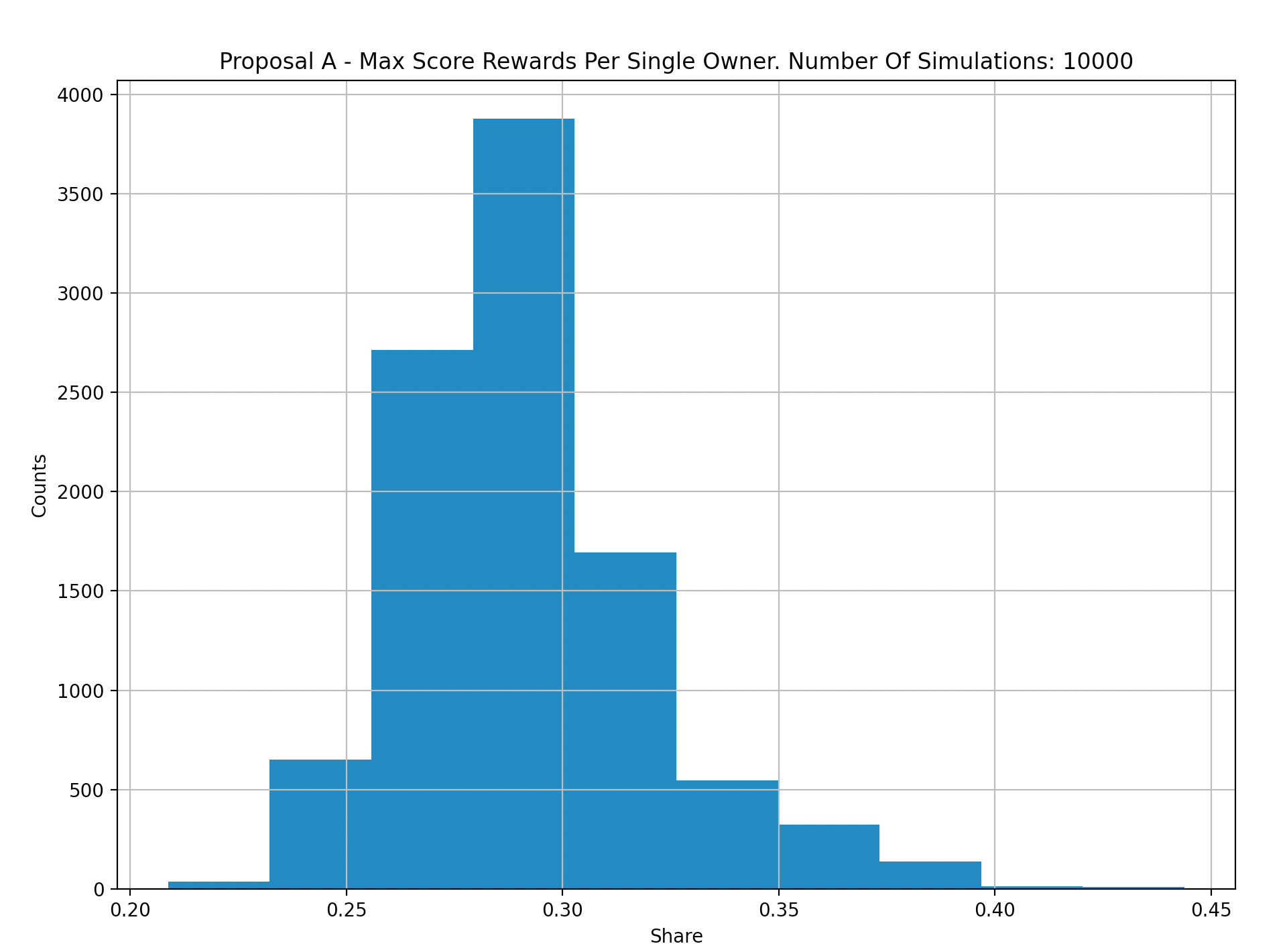
X-Axis: Max Weighted Score for a single maker.
Y-Axis: Count per 10k simulations.
Based on our simulations, we observe that G-score reduces the likelihood of a single top performer obtaining the majority of rewards. However, as our analysis of historical data has shown, this is not always the case in reality. Therefore, we plan to refine our "Gamers" market maker models to more closely resemble real-world behavior.
Furthermore, we find that the Weighted-Score modification reduces the likelihood of a single user obtaining over 40% of rewards in the simulated input data compared to the G-score modification, which had 50-60% in rare but possible cases. This finding supports the conclusion that the Weighted-Score modification is preferable to the G-score alternative.
G-score, Weighted-Score versus Q-score based on volume fairness
To facilitate understanding of the data, we divided the simulation samples into batches of 50 and created graphs to illustrate the maximum/minimum rewards multiplier versus volume. A multiplier of 1 indicates that the rewards are in a 1:1 proportion to the volume of a particular LP, while a multiplier of 2 indicates that the LP received rewards that are twice as large as their share in the volume.
It is important to note that the following presentations are in log scale, which makes it easier to visualize the gaps.
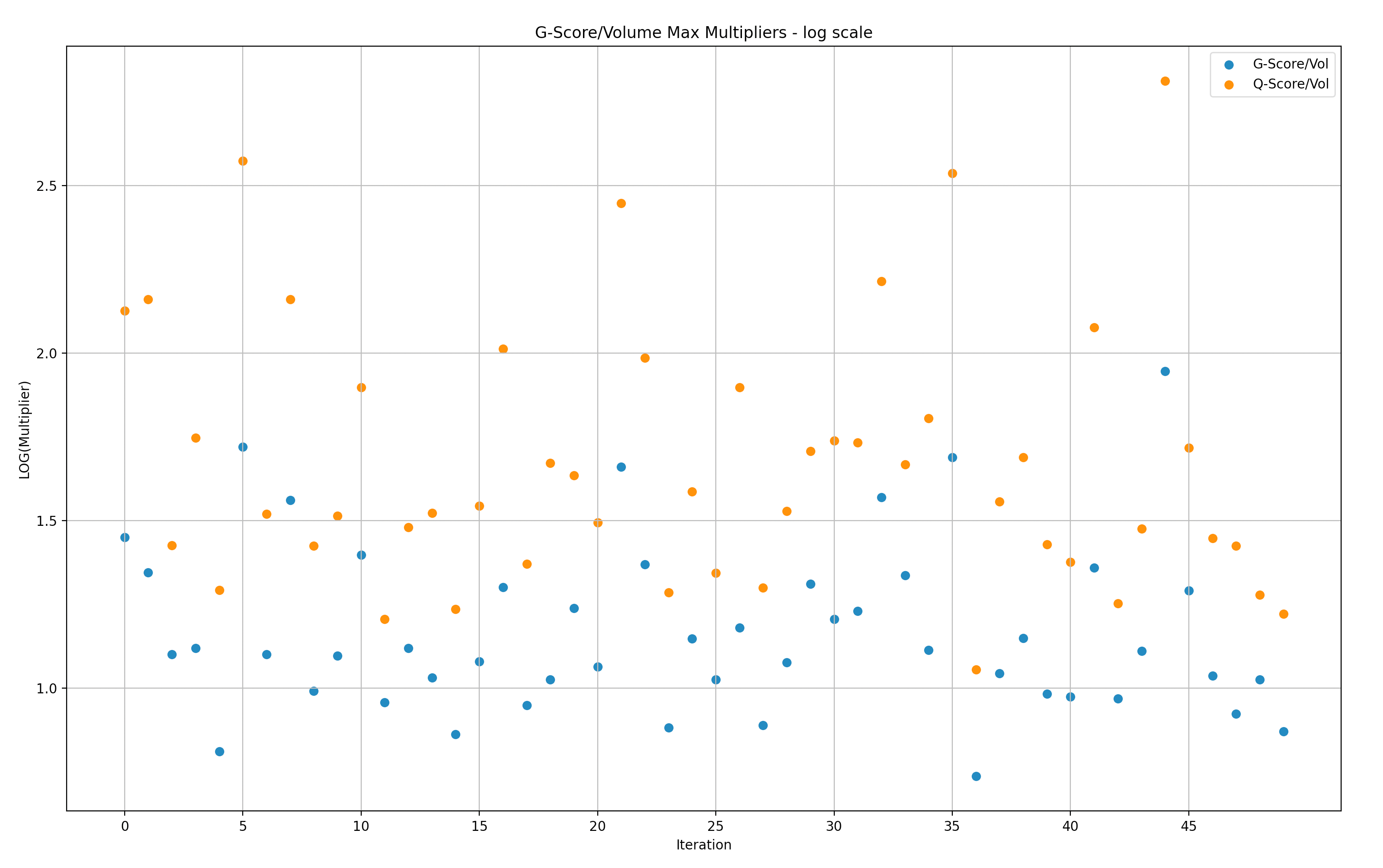
Y-Axis: Max G/Q score over volume in Log scale(multiplier of rewards versus Volume)
X-Axis: simulations (50)
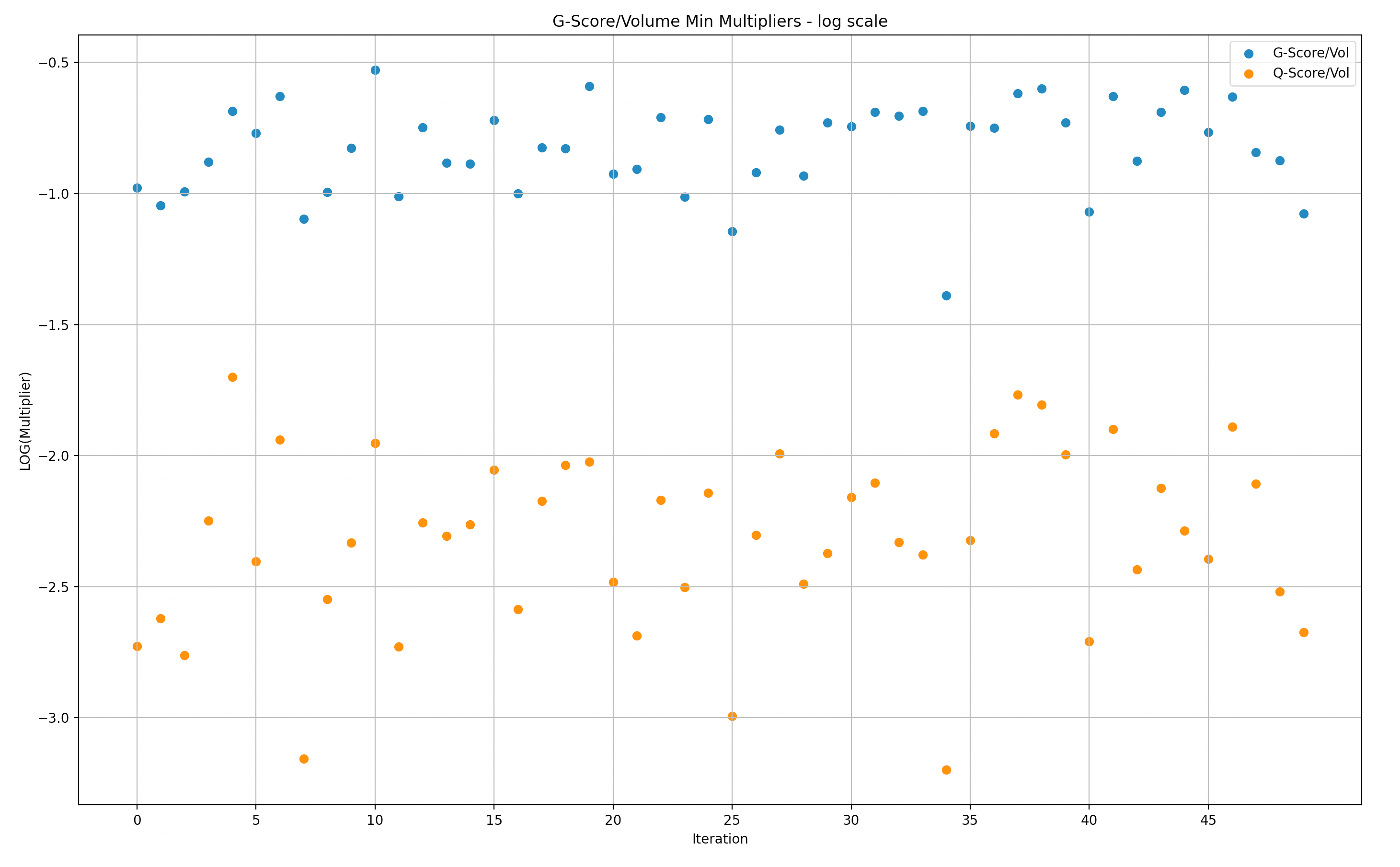
Y-Axis: Min G/Q score over volume in Log scale(multiplier of rewards versus Volume)
X-Axis: simulations (50)
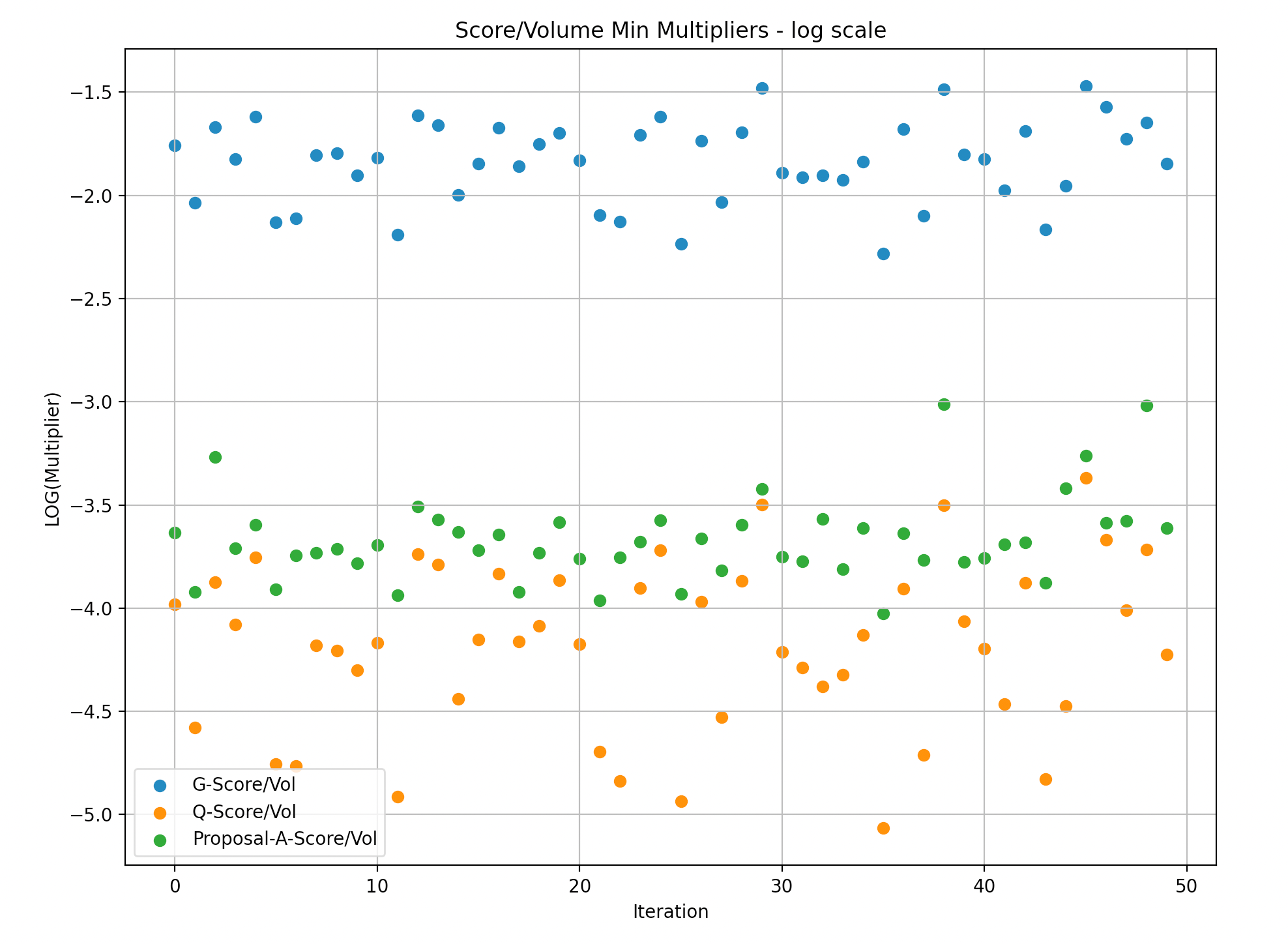
Y-Axis: Max G/Q/W score over volume in Log scale(multiplier of rewards versus Volume)
X-Axis: simulations (50)
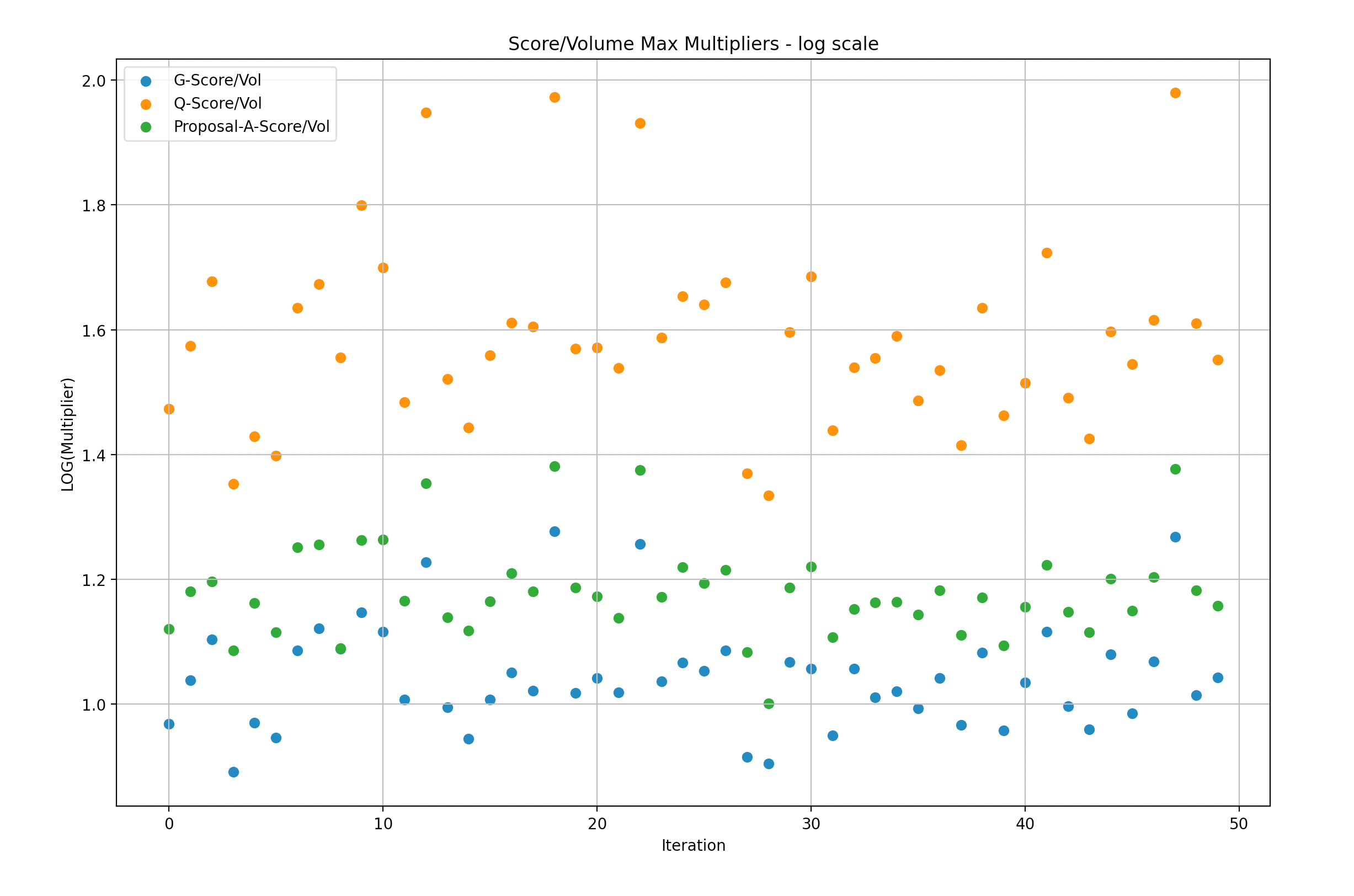
Y-Axis: Min G/Q/W score over volume in Log scale(multiplier of rewards versus Volume)
X-Axis: simulations (50)
G-Score versus Q-Score
We narrowed the simulation samples to batches of 50 in order to expose the data in a way that is more digestible.
Similar to the data above for volume, these represent the data in relation to Q-score instead of volume. Also in log scale.
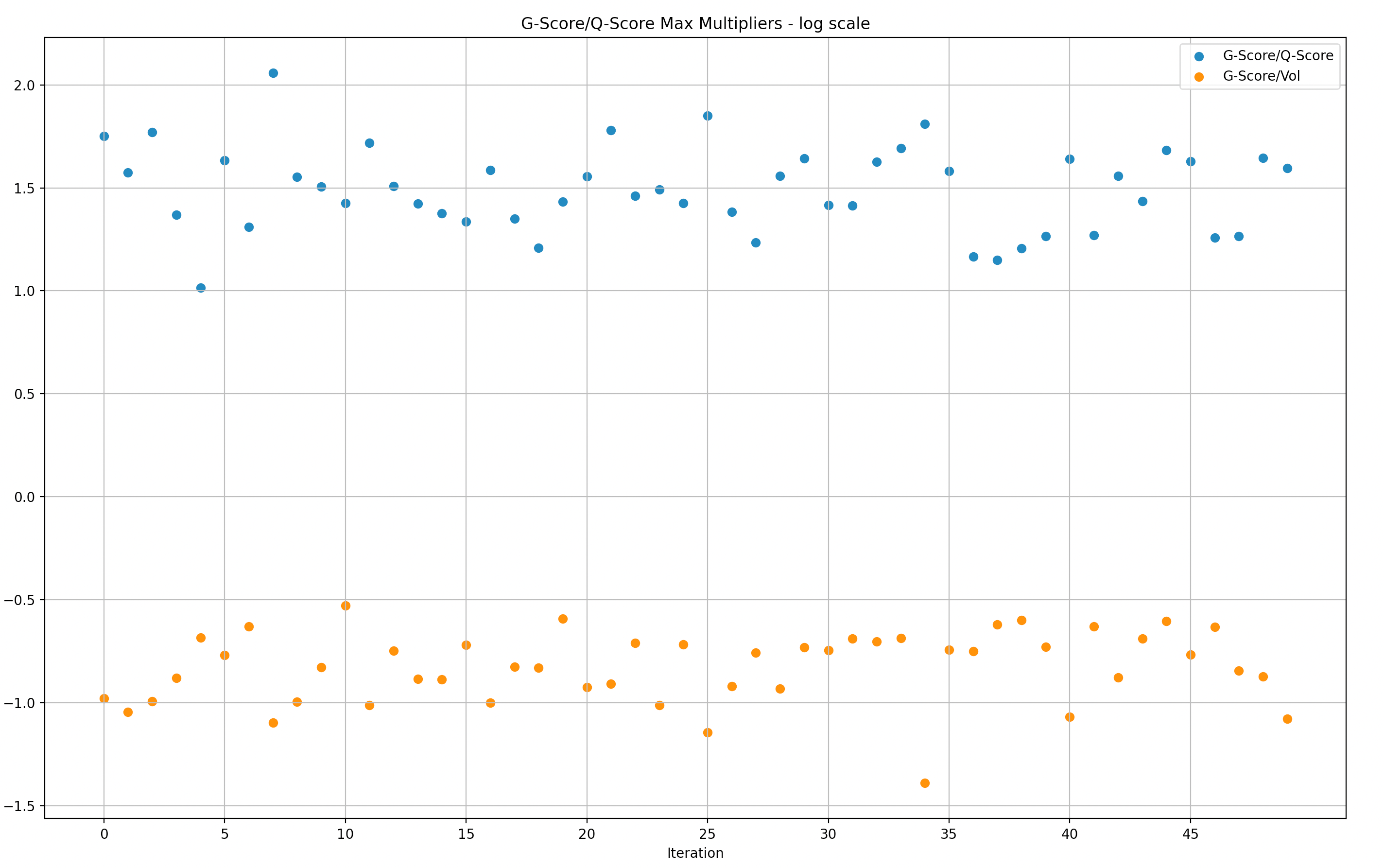
Y-Axis: Max G-score over Q-score in Log scale(multiplier of rewards versus Q-score)
X-Axis: simulations (50)
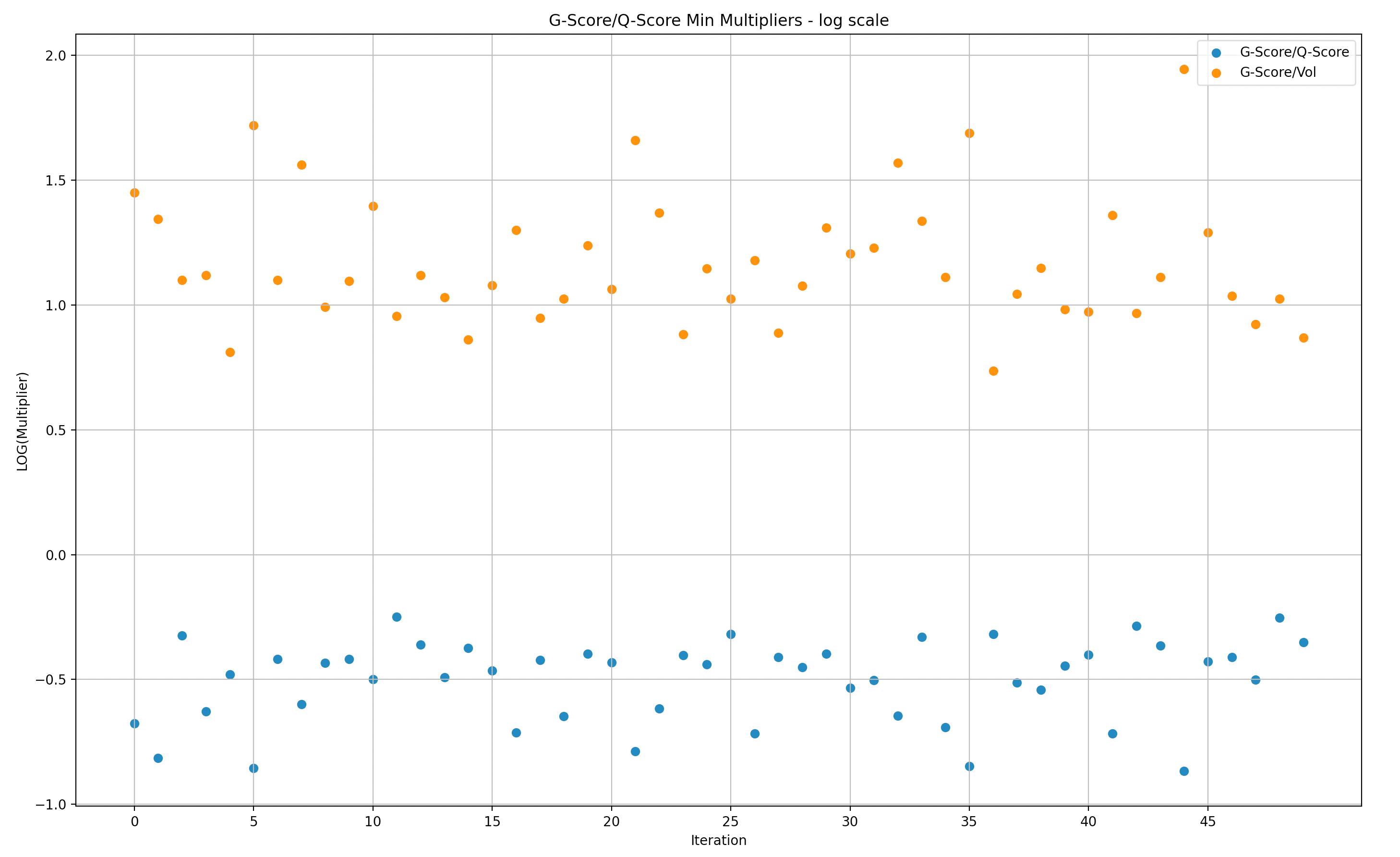
Y-Axis: Min G-score over Q-score in Log scale(multiplier of rewards versus Q-score)
X-Axis: simulations (50)
To give better context to the data above, we can see the specific top scorers that generated the ratios order in max and then minimum.
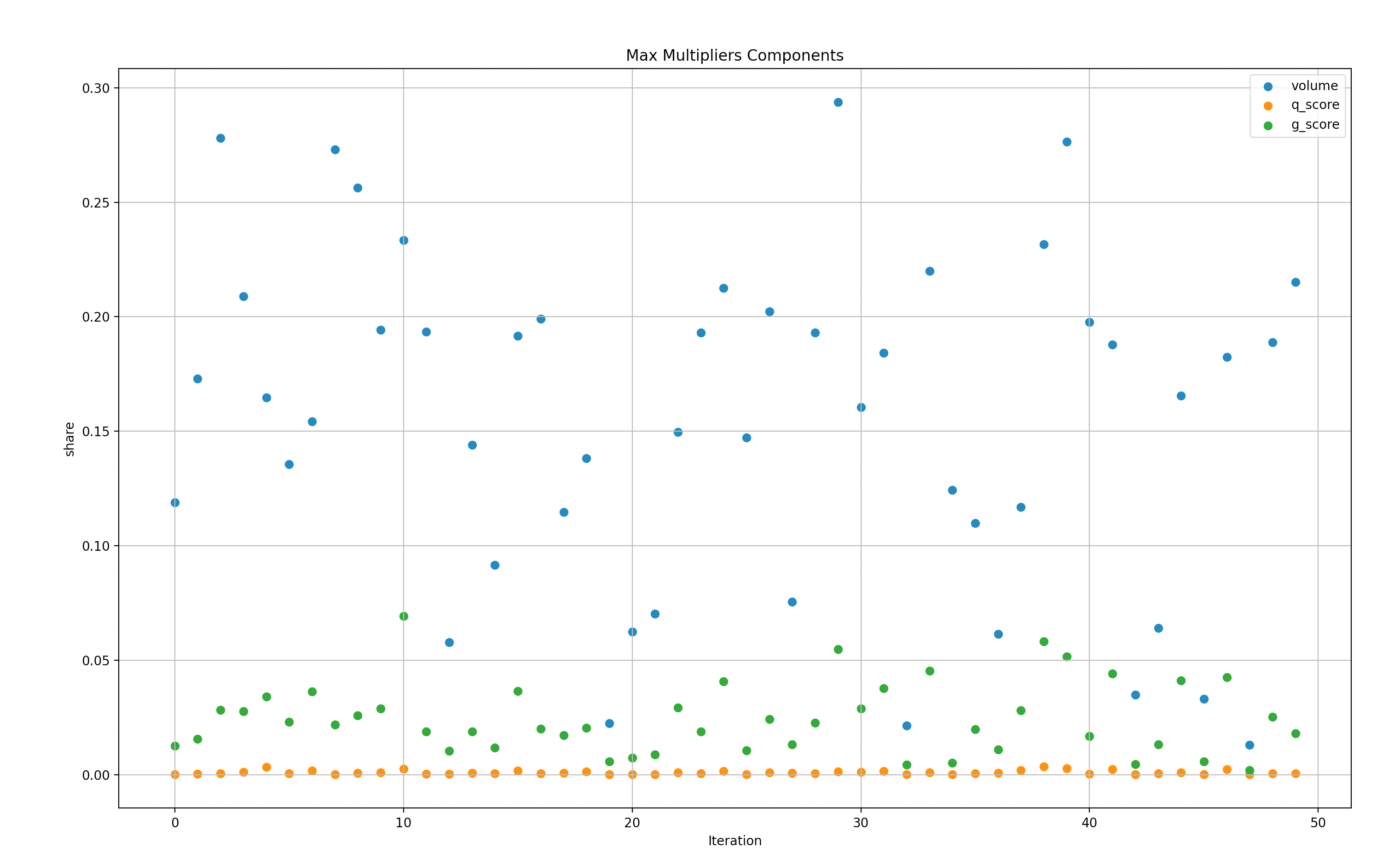
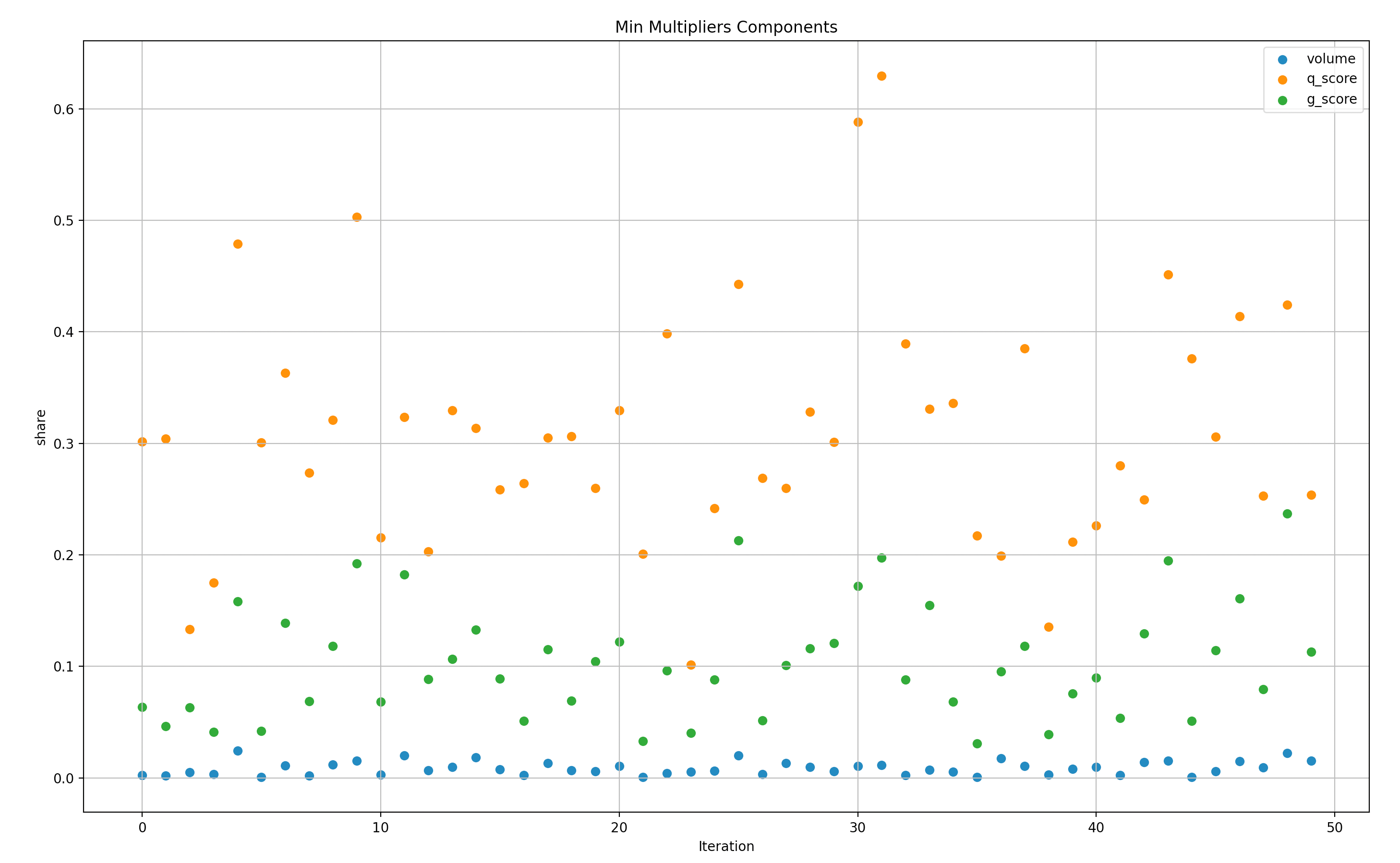
Simulations Result Summary
Based on our analysis of the simulation results it is clear that Weighted-Score/G-score is an improvement to the Q-score formula and more difficult for individual makers to manipulate. Most of the time Weighted-Score vs G-score show similar performance, however, Weighted-Score seems to perform better at mitigating disproportional single user rewards for the same simulation data sets, even when having either a disproportional Q-min score or volume share.
It is notable that there’s still work to be done to improve the efficiency of the Weighted-Score/G-score, minimizing the gaps between the Q-score and volume multipliers to the rewards. There are also risks that require further research and remediations in future iterations. These simulations, while powerful, don’t fully address (yet) these potential issues.
We have tried to highlight the “low-hanging” risks in this analysis, but in an ever-changing market, continuous review and analysis will be required to ensure that the formula and distribution strategy survive the test of time.
Next Steps
The above represents the tip of the iceberg in what research the Chaos Labs team intends to complete under this proposal. These simulations have been run to catch what is most obvious in terms of ways the equations could be gamed but have not begun to scratch the surface of potential risks.
We believe that with more time, Chaos Labs would be able to provide a more thorough analysis of the shortfalls of current proposals in addition to a newly proposed solution with a minimal surface area of attack.
Once a new equation is approved by the community, we will use these same simulations in addition to new market data to continue to stress test the LP rewards incentivization scheme to be most efficient at its stated goals: tightening spreads and competition on volumes.
Specific work we intend to do:
- Optimize to optimal weights for the future formula to achieve the fairest distribution according to the desired metrics.
- Review additional vulnerabilities/attack vectors in Q-score & G-score or Weighted-Score.
- Research and iterate to formulate more resilient scoring mechanisms for fairer and tamper-proof formulas
- Further explore situations of incentive misalignment (underpaying “good” makers, overpaying manipulators, etc.)
- Ongoing monitoring and adjustments to stay one step ahead
About the dYdX Grant Program
If you want to learn more about the dYdX Grants Program, check out their blog.