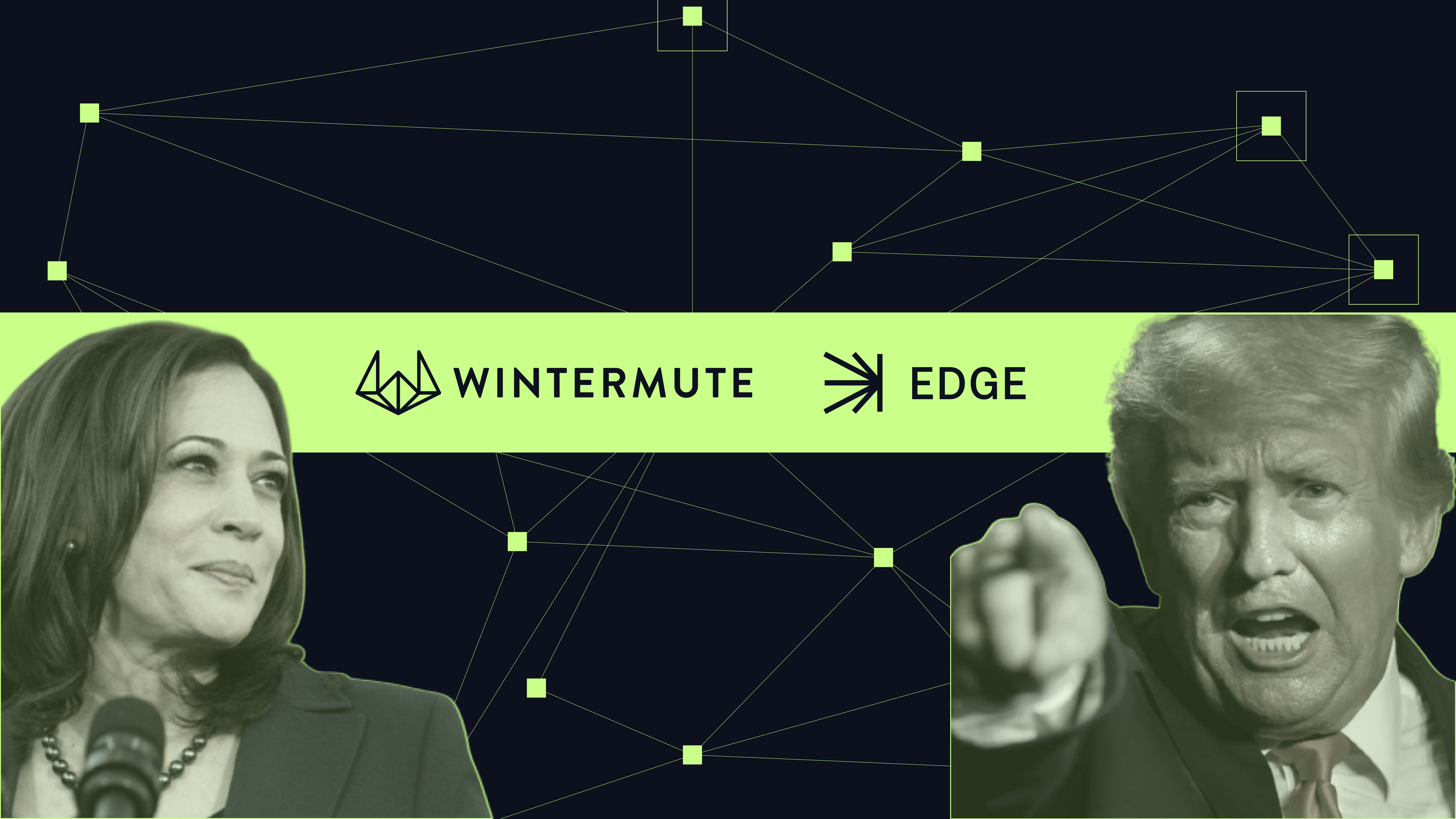
A New Era for Prediction Market Oracless
Prediction markets, such as those for the US elections, are revolutionizing how we view real-world events. At Chaos Labs, we’re excited to announce the launch of Edge Proofs Oracles.
Wintermute, a leading global algorithmic trading firm, has selected Edge Proofs Oracles to power OutcomeMarket, its new U.S. Presidential Election prediction market. The market will utilize Chaos Labs' Edge Proofs Oracle to guarantee the highest precision in data delivery across multiple chains.
Edge Proofs Oracles ensure verifiable data provenance, integrity, and authenticity, enabling blockchain applications to trust the external data they rely on. This capability is crucial for Prediction Market Oracles, a specialized subset of proof oracles designed to bring off-chain data on-chain in a secure and trusted manner, ensuring accurate verification of real-world outcomes like elections.
The process of a Prediction Market Oracle works as follows:
- Declare Reputable Sources: Trusted sources like the Associated Press, CNN, or Fox are designated in advance. These serve as the inputs for resolving prediction market events, such as determining the winner of a U.S. election.
- Prove Data Provenance, Integrity, and Authenticity: The Oracle ensures that the data remains in its original form, untampered, and precisely as published by the declared sources, preserving the integrity of the input.
- Determining Event Outcome: Advanced AI or LLM models process the text and generate insights that answer specific questions, such as "Who won the U.S. election?" without adding subjective interpretations.
- Achieve Consensus: To ensure the reliability of the outcome, the Oracle network achieves consensus between multiple nodes. This step guarantees that no single entity can unilaterally determine the event's outcome, ensuring transparency and decentralization.
Crucially, Prediction Market Oracles do not act as arbiters of truth but ensure that trusted data sources and transparent methods are used to resolve event outcomes. This approach delivers a scalable, decentralized solution while minimizing human bias and intervention.
This post excavates the critical challenges facing prediction markets—proving data provenance and verifying data accuracy—and how Edge Proofs Oracles address these challenges using AI models such as LLMs. Let’s explore the challenges and our approach to addressing them.
Why Data Provenance Verifiability is More Important Than Ever
Data provenance refers to the ability to trace and verify the origin and history of data. In decentralized systems, ensuring authenticity means that data has come from a trusted source and has not been altered during transmission.
For blockchain applications—especially those like prediction markets—the accuracy of inputs directly influences outcomes. If the source of the data is not verifiable or tampered with, it can lead to inaccurate decisions or financial consequences. Ensuring the data’s provenance and authenticity can be cryptographically verified is crucial to maintaining trust in decentralized systems.
We Are in a Trust Crisis
We are in a trust crisis; we no longer know who to trust and do not have a clear picture of where data emanates from and whether it is reliable.
With the rise of generative AI and LLMs, the cost of producing high-fidelity fake content is approaching zero, making it harder to distinguish fact from fiction. Fraudulent information can now be created, altered, and erased with minimal effort, eroding digital trust.
Chaos Labs is directly addressing this challenge with the Edge Proofs Oracle for Prediction Markets, which will power OutcomeMarket, Wintermute’s 2024 US Election Prediction Market. Verifying the authenticity and origin of data is essential—not just for human decision-making but also for autonomous agents and decentralized systems. These systems rely on data whose provenance and integrity are not verifiable through protocols like HTTPS.
Verifiable data is not just a theoretical concern; it's a critical issue in an increasingly trust-dependent world where unreliable information is more prevalent than ever.
The Danger of Revisionism
The Internet's mutability creates an opportunity for revisionism. Once public, content can be quietly altered or erased, leaving little to no trace of the original material. Websites and publications can change the facts of their reporting without accountability, resulting in a lack of integrity and increasing public mistrust in media.
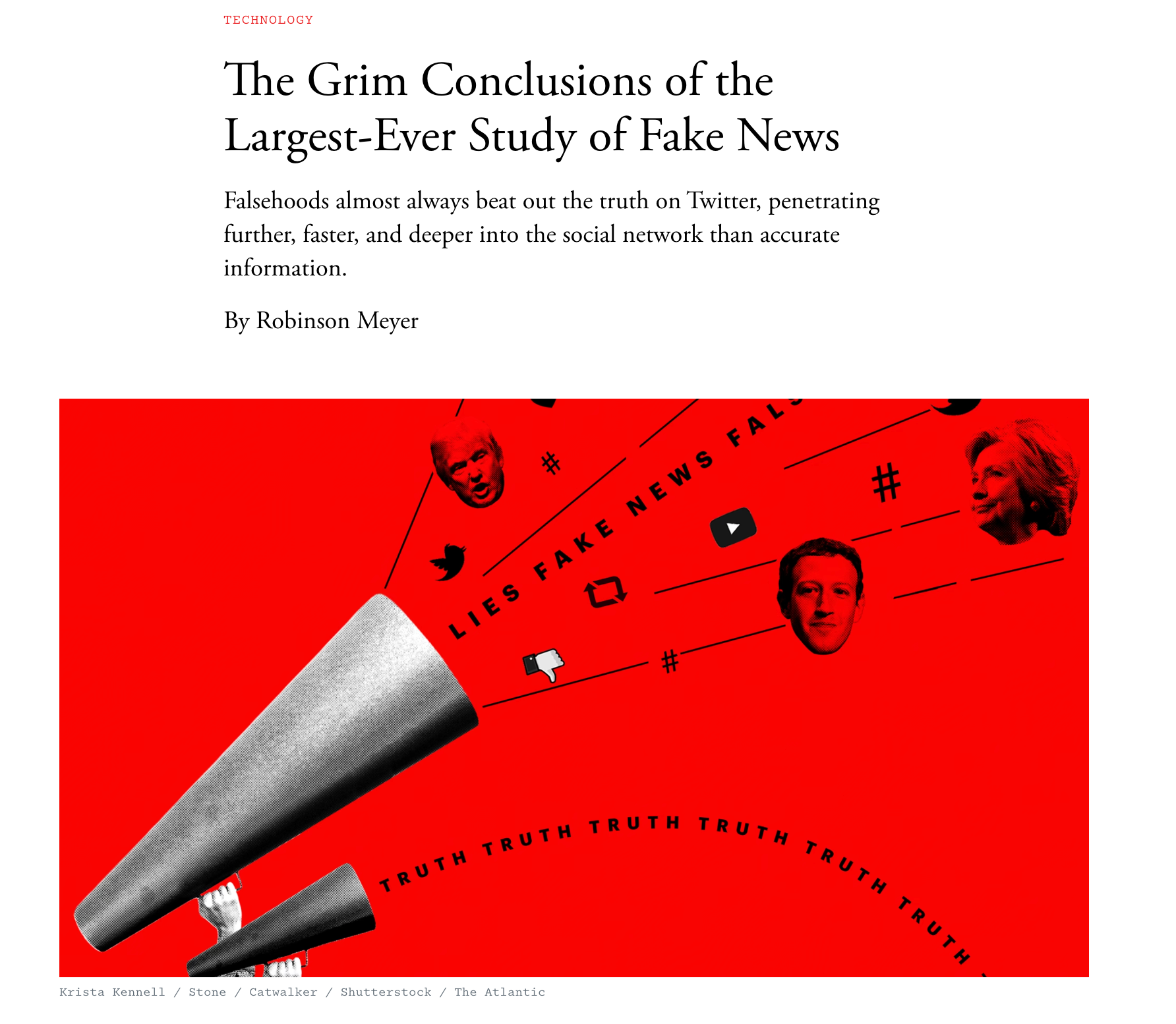
There is no canonical immutable record of what was published, and no system guarantees a piece of information is as it was initially posted.
Humans Eroding Trust
Initiatives like the Wayback Machine aim to serve as a record of the internet by capturing snapshots of websites, preserving their content even if it changes or is deleted. It helps solve problems like archiving web history and information deletion.
However, it’s not perfect. It misses some web pages due to exclusions (like robots.txt
), doesn’t handle dynamic (JS-rendered) content well, and has limited resources, meaning it can’t archive everything. Server issues and infrequent crawls can also cause gaps in its records.
Despite these limitations, it’s a valuable resource for preserving the web’s history.
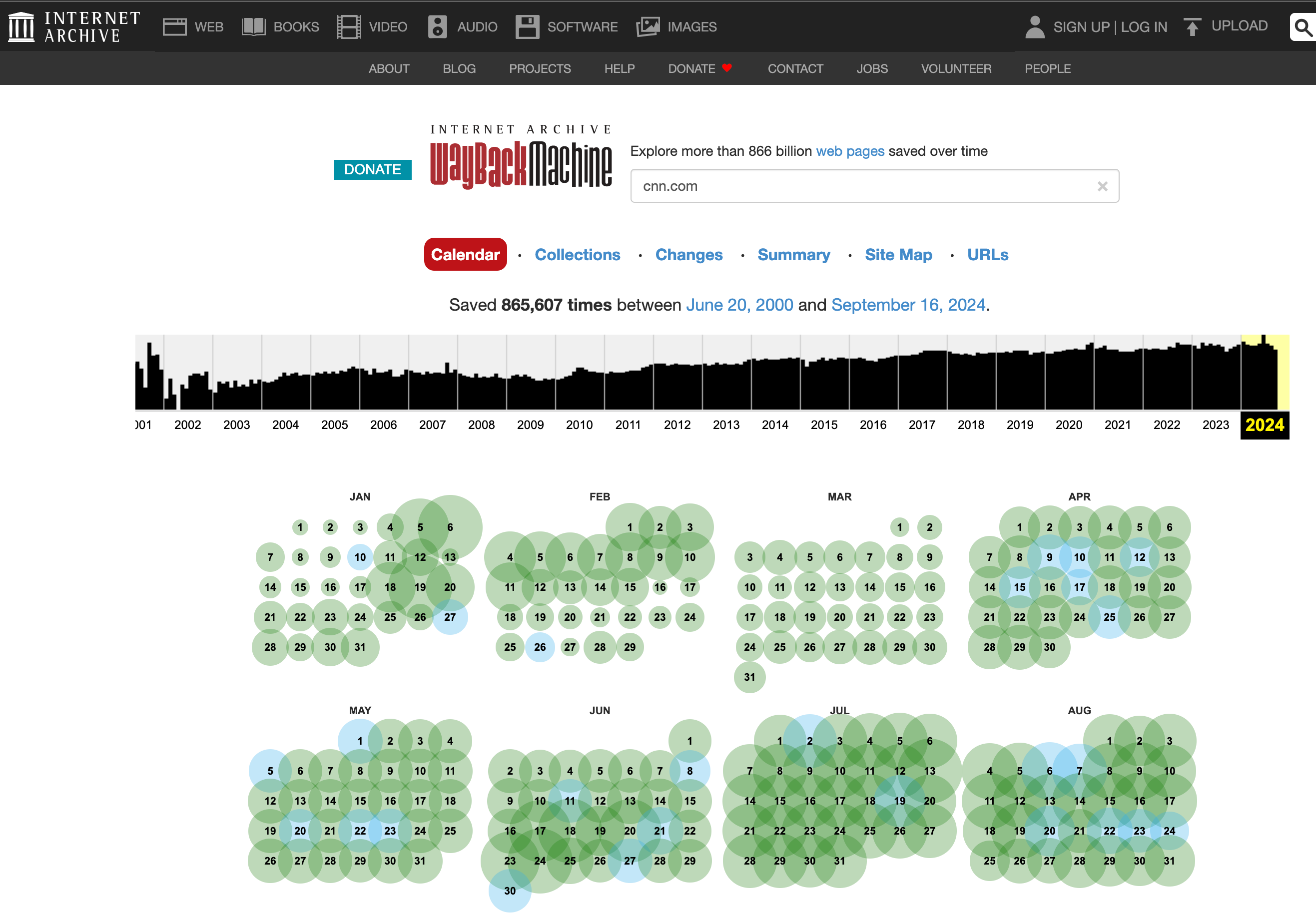
Also, as we’ll see below, unveiling the truth can be extremely difficult. Are Haitian migrants eating pets in Springfield? Did the US fake the lunar landing?
Determining Event Outcomes
Currently, determining event outcomes in prediction markets is often handled manually. Human participants or centralized entities analyze available data, weigh sources, and vote or declare outcomes based on their interpretations. This manual process, while straightforward, introduces the possibility of human bias, conflicting incentives, and subjective interpretations.
Using LLMs (Large Language Models) like GPT for prediction markets offers a streamlined alternative. When tasked with confined, well-defined scopes—such as answering whether candidate A or B won an election based on a "hard news" article—LLMs excel at parsing data efficiently and consistently. For instance, an LLM can process factual news content published by trusted outlets like CNN or the Associated Press to deliver clear and accurate responses, such as confirming the winner of an election once the results are officially declared by the aforementioned publications.
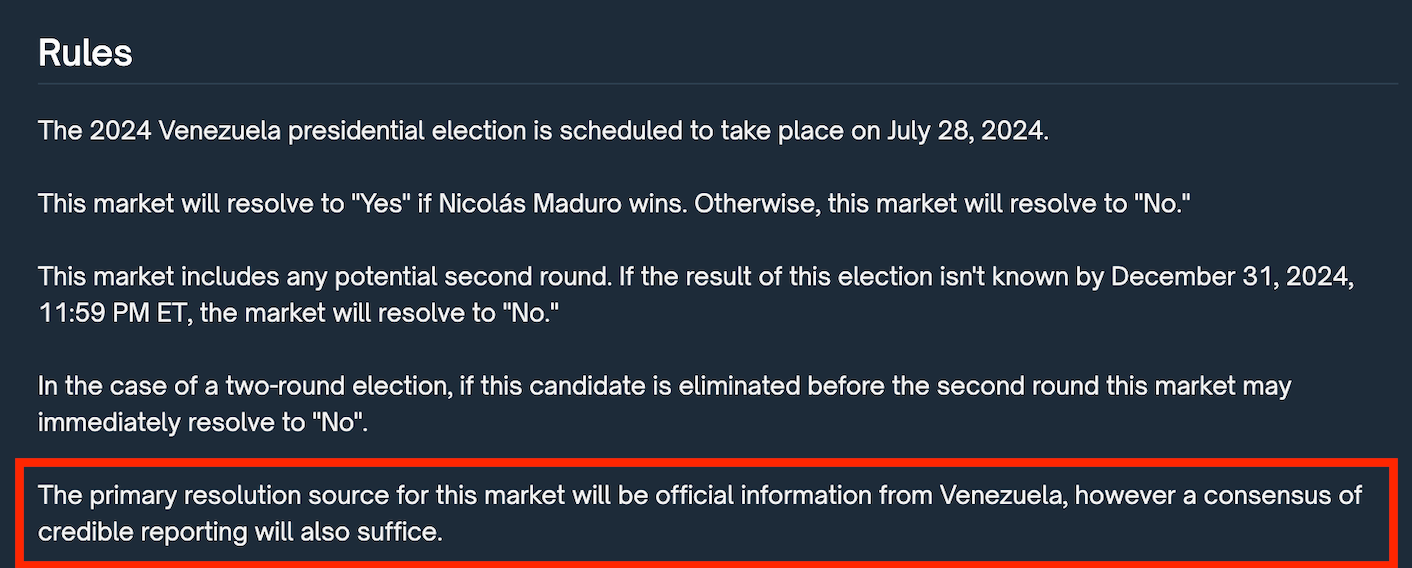
However, it’s crucial that prediction market prompts be clear and unambiguous. Poorly defined prompts can lead to confusion, as was the case with the 2024 Venezuelan Election market on Polymarket. The prompt raised questions about what constituted "official information" from Venezuela and what counted as a "consensus of credible reporting." The lack of clarity allowed for multiple interpretations, creating uncertainty for both AI models and human participants. In order for LLMs to determine outcomes reliably, explicit rules and well-scoped tasks are essential, minimizing the potential for ambiguity.
A Case Study: Oracle Performance During the 2024 Venezuelan Election Prediction Market
The 2024 Venezuelan Election Market on Polymarket serves as a stark example of the complexity of resolving real-world events in decentralized prediction markets. As traders bet on the outcome, the market shifted from reflecting predictions to actively shaping the narrative, revealing just how difficult it can be to verify the truth in politically charged situations.
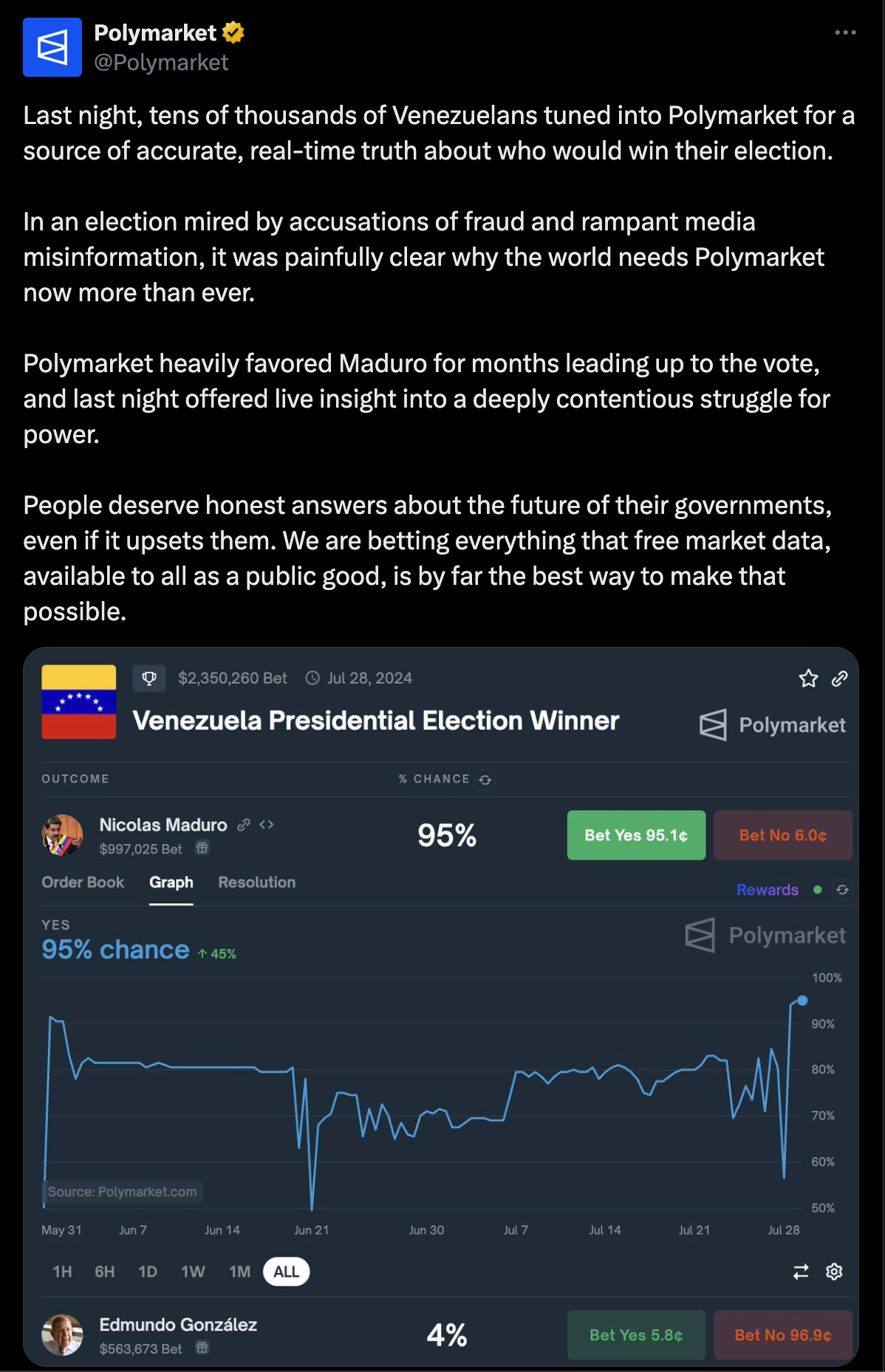
The market initially leaned toward Nicolás Maduro as the winner, supported by official election results from the Venezuelan Electoral Commission (CNE). However, this wasn't the end of the story. The opposition refused to concede, so they claimed victory and provided their own data to back it up.
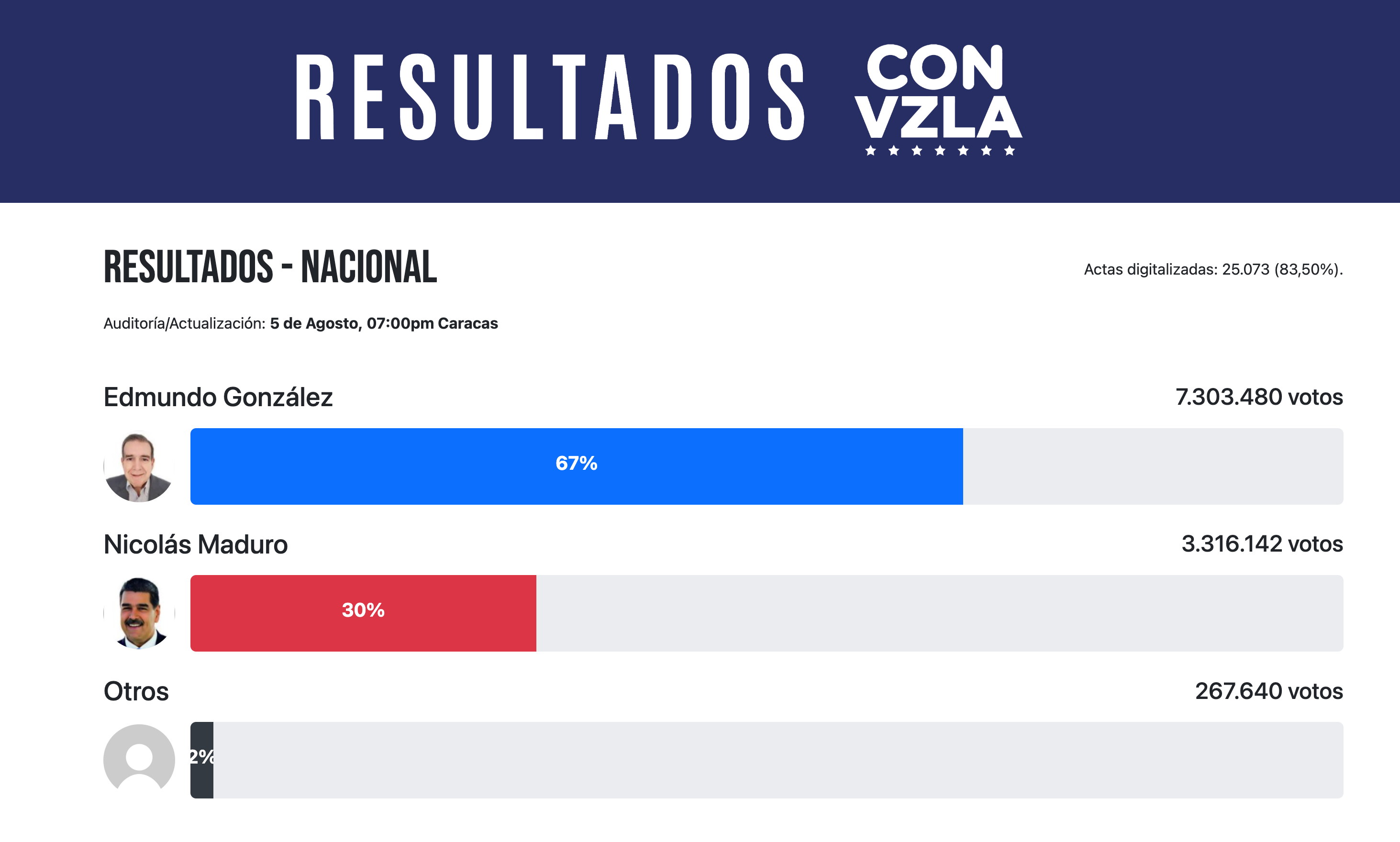
This created an environment of intense uncertainty, with conflicting narratives making it difficult to determine which outcome was real.
At the center of the market resolution was UMA Protocol, an optimistic, human intervention-based oracle and the oracle responsible for determining the market outcome. UMA voters had to make a decision amidst the chaos, choosing between the official data provided by the Venezuelan government and the opposition’s digital counter-narrative. In the end, UMA resolved the market in favor of the opposition candidate, González, despite the global consensus favoring Maduro. This decision underscored a critical issue: when the outcome of a prediction market rests on the oracle, the oracle ceases to be a passive reporter of facts and instead becomes a powerful arbiter of truth.
This situation illustrates the immense difficulty of resolving real-world events in decentralized systems, particularly in contentious political environments. Oracles like UMA rely on human decision-making, leading to questions about manipulation, bias, and centralization of power. Traders and observers were left to wonder whether the oracle had merely reported or determined the outcome.
The Venezuelan election market on Polymarket highlighted a complex issue: how ambiguity in a prediction market prompt can lead to confusion for voters and leave room for exploitation. In this case, the market's resolution criteria — “official information from Venezuela” and “a consensus of credible reporting” — were imprecise. This ambiguity forced both voters and oracles to interpret what constitutes “credible reporting,” leading to conflicting views and the potential for manipulation. The role of the oracle, originally meant to observe and resolve, became a central factor in shaping the market itself.
This situation underscores the difficulty of resolving prediction markets with human intervention under uncertain or contested conditions, further complicating the idea of neutrality. At Chaos Labs, we've taken these lessons to heart while developing the Edge Proofs Oracle for the 2024 U.S. Election Prediction Market. Our approach leverages LLMs to eliminate human bias, reducing the risks of centralized control, manipulation, and subjective decision-making. However, precise prompts and clear rules are crucial to maintaining the integrity and fairness of prediction markets.
LLM Powered Prediction Market Oracles
Relying on verifiable machine learning models for oracle solutions offers advantages over human-driven voting systems, particularly in high-stakes environments where trust, accuracy, and efficiency are paramount.
Removing Human Bias and Ensuring Accuracy with Data-Driven Models
Human-driven systems are prone to biases stemming from personal beliefs, political leanings, or financial incentives. In prediction markets, this introduces a risk of manipulated or skewed outcomes. Emotions like fear or greed can further influence decisions, especially when the stakes are high and objectivity is crucial.
In contrast, machine learning models process data systematically according to predefined rules. While these models are not entirely free from bias—since their outputs depend on the quality and diversity of their training data—they can be rigorously tested and refined. ML models, when trained on fair data, offer a more structured approach that minimizes external influence, promoting objectivity and fairness.
Moreover, the ability to benchmark, validate, and refine ML models makes them highly effective in delivering consistent results, especially for straightforward, well-defined tasks like verifying election outcomes from trusted news sources. Unlike human-driven systems that can vary in interpretation, ML models follow the same logic and produce repeatable outcomes when given the same inputs. This structured, data-driven approach is key to ensuring accuracy and reliability in resolving prediction market events.
Verifiability and Transparency
In human systems, it’s difficult to trace the reasoning behind each vote or decision. Even when outcomes can be audited, understanding the motivations behind decisions remains opaque. This lack of transparency undermines trust.
By contrast, ML models are fully verifiable. The training data, model parameters, and inference processes can be audited, making the entire decision-making process transparent. Every prediction can be tracked back to its inputs, allowing third parties to verify outcomes with clarity and confidence.
Speed, Scalability, and Automation
Machine learning models are highly scalable and can process enormous amounts of data in real-time. Where human systems require deliberation, coordination, and slow voting processes, ML models deliver results almost instantly, making them perfect for environments that demand speed and efficiency.
Additionally, ML-based Oracles can be automated. They don't require human intervention at every step, which dramatically reduces operational overhead and increases throughput. Whether handling large transaction volumes or processing complex data from multiple sources, ML models can operate autonomously without losing accuracy.
Resistance to Market Manipulation
Human-driven voting systems are susceptible to manipulation, especially when individuals have significant financial stakes in the outcome. Large market participants may attempt to sway results through coercion or financial incentives, introducing substantial trust issues—particularly in decentralized systems.
Machine learning models, however, are resistant to such manipulation. These models make decisions based on predefined logic and data rather than external pressures or incentives. As they do not participate in the markets themselves, and have no financial positions, they are immune to conflicts of interest. By removing humans from the decision-making process, ML models greatly reduce the risk of tampering or undue influence, ensuring that prediction markets remain objective and trustworthy.
Wait, so are LLMs a Silver Bullet?
While LLMs (Large Language Models) hold great promise, they are not a one-size-fits-all solution. It’s important to acknowledge their limitations. One recent example is the Google Gemini model, which, in some instances, was found to rewrite parts of American history, highlighting the fact that LLMs can sometimes produce flawed or inaccurate responses.
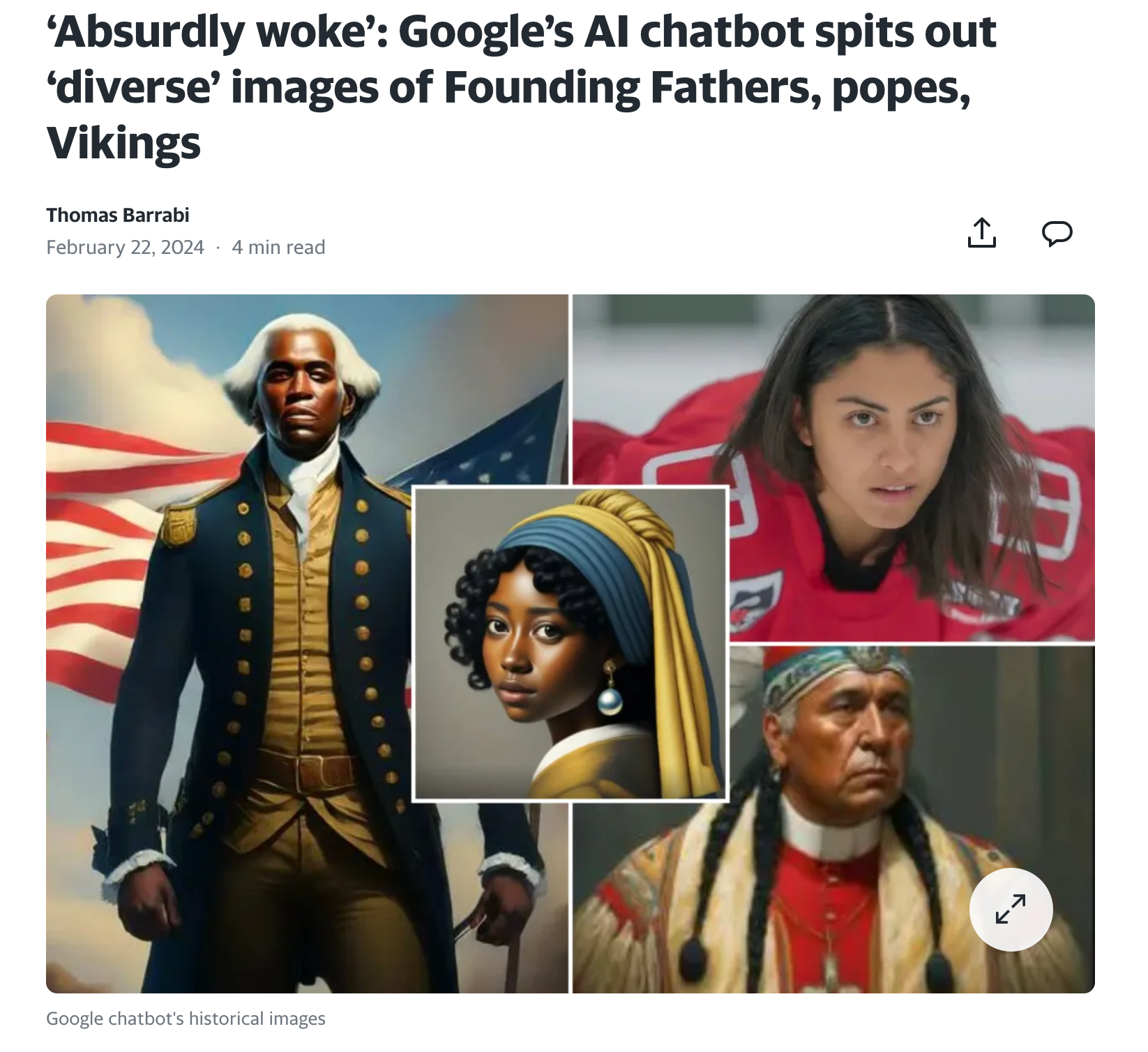
These shortcomings stem from biases in the training data and the inherent variability of language models.
That said, when it comes to prediction market oracles that require straightforward answer extraction from well-defined and authoritative sources—such as determining the winner of an election based on articles from reputable news outlets like CNN or Associated Press—we believe LLMs can still be highly effective. The key lies in focusing the model on simple tasks that revolve around factual, declarative statements. For example, when CNN publishes an article declaring the winner of an election, the LLM’s job is simply to extract the explicit statement of the winner—nothing more.
This controlled, limited-scope environment minimizes the complexities that typically introduce variability in LLM outputs. The LLM isn't being asked to interpret or weigh subjective data; it's just pulling out clear, factual declarations. This simplicity helps minimize the potential for error that could arise in more abstract or open-ended tasks.
However, we’re under no illusion that this system is perfect. LLMs are still evolving rapidly, with each iteration bringing improvements in reasoning ability and general intelligence. As pioneers in LLM-based Prediction Market Oracles, Chaos Labs is taking a cautious, methodical approach. We’re employing safeguards and oversight mechanisms to ensure that while the technology matures, its use remains safe, reliable, and transparent. By acknowledging the current limitations and preparing for rapid future advancements, we believe LLMs will play a significant role in the future of decentralized prediction markets.
What's Next
In the coming days and weeks, we will release a series of technical deep dives into our approach to solving the key challenges of data provenance, authenticity, and LLM-based outcome determination. These deep dives will break down the mechanics behind Edge Proofs Oracles, explaining how we ensure trust in off-chain data brought on-chain. We’ll walk through the cryptographic proof mechanisms we employ to verify the source and integrity of external data and detail the design choices that allow us to utilize LLM technology for outcome extraction in prediction markets. Stay tuned!