Introduction
Reference chapters:
- Oracle Risk and Security Standards: An Introduction (Pt. 1)
- Oracle Risk and Security Standards: Network Architectures and Topologies (Pt. 2)
- Oracle Risk and Security Standards: Price Composition Methodologies (Pt. 3)
- Oracle Risk and Security Standards: Price Replicability (Pt. 4)
- Oracle Risk and Security Standards: Data Freshness, Accuracy, and Latency (Pt. 5)
The previous chapter on Oracle Network Architectures and Topologies examined the critical structures that underpin oracles. It outlined the complexities of data flow within these networks and the security and risk challenges they face.
This chapter delves into the intricate world of Price Composition Methodologies, a foundational pillar of Oracle risk and security. It encompasses an in-depth analysis of data source selection and validation, an exploration of the limitations of rudimentary pricing methods and opportunities for improvement, concluding with an examination of the tradeoffs between price aggregation techniques grounded in real-world examples. This holistic overview guides readers through the practices and innovations that exemplify the relationship between price composition and Oracle security.
To begin we examine the assumptions and principles guiding price composition, highlighting that the definition and objective behind pricing assets are inextricably linked to their specific use case and the utility they deliver to the end user.
Price, which Price?
Given that assets often trade simultaneously on multiple venues, there can be many possible prices for an asset at any point in time. So, how does one determine the true price? This question is perhaps best answered by exploring the prevailing DeFi use cases for which a price is required.
In DeFi, there are two predominant use cases:
a) Pre-trade pricing, whereby a swaps platform would price each trade request separately given where the market is (the market is defined as the total collective of liquidity sources outside of the platform itself).
b) Post-trade pricing, whereby a platform and its users would periodically mark-to-market their positions, assets, or value of their collateral.
From a price measurement perspective, both use cases can be satisfied with a price that emulates a “Best Execution” price (ft 1), which represents the best price that can be achieved (buy or sell) through liquidity sources available in the market. For the use case, a) it is a price that enables the swap to remove the need to trade elsewhere on multiple venues simultaneously, and b) the price represents the value at which the asset can be liquidated at the time of the price measurement.
Composing such a price underscores the importance of meticulously selecting exchanges and liquidity sources, defining the Relevant Market (ft 2), and the need for continuous market data monitoring.
Footnotes
- Best Execution is a concept formally defined for equities by regulatory regimes like MiFID II. It optimizes for order execution prices and likelihood of execution for end-users.
- Relevant Market is a term used to describe the collective of liquidity sources that are robust, transparent, and reflective of true market conditions. It involves selecting venues that provide the most accurate representation of an asset's price at any given time, based on liquidity, trading volume, and the absence of manipulative practices.
Data Sourcing and Validation
The quest for pricing accuracy is not merely an academic pursuit but a practical necessity, grounded in selecting exchange and liquidity sources that are robust, transparent, and reflective of true market conditions. Unlike traditional markets, there is no equivalent to a consolidated tape that provides a unified view of transactions across exchanges. This creates a complex environment where data consistency and reliability are hard to guarantee, impacting decision-making and market transparency. This section examines the critical process of selecting these sources and the rigorous criteria they must meet to be considered part of the Relevant Market — a concept at the heart of trustworthy price composition.
Transparency
Oracles should transparently disclose their methodologies for defining the Relevant Market. Such disclosure not only enhances the credibility of the price data but also ensures that stakeholders clearly understand the data's foundation. In evaluating potential sources, the focus extends beyond mere price points to a holistic assessment of exchange and instrument quality. Given the significant disparities among exchanges, particularly in crypto, our approach emphasizes ongoing monitoring and validation across several key dimensions.
Price Breadth
To improve the robustness of the price, it is critical to expand the range of high-quality liquidity sources from which it is constructed. This involves highly liquid markets that may use a variety of base assets, including other fiat currencies, stablecoins, and blue-chip crypto assets. For example, the XRP-USD price may be more robust when incorporating deeply liquid XRP-KRW instruments. However, this also introduces challenges, namely managing cross-rate risks. To address these issues, oracle providers must perform triangulations to maintain accurate exchange rates across different currency pairs and monitor for anomalies like stale or deviant rates, ensuring that the pricing remains representative of true market conditions.
Exchange Evaluation Framework
A robust exchange evaluation framework should encompass several key criteria:
- Volume
- Access to market data
- Technical and security robustness
- Policies and effective monitoring of illegal, manipulative, or unfair trading practices.
Volume
The process of selecting high-quality exchanges for inclusion in the Relevant Market hinges primarily on the analysis of exchange volume, emphasizing the importance of substantial and legitimate trading activity as an indicator of healthy liquidity. Robust, organic trading volume suggests that an exchange is trusted by users and plays a role in price discovery.
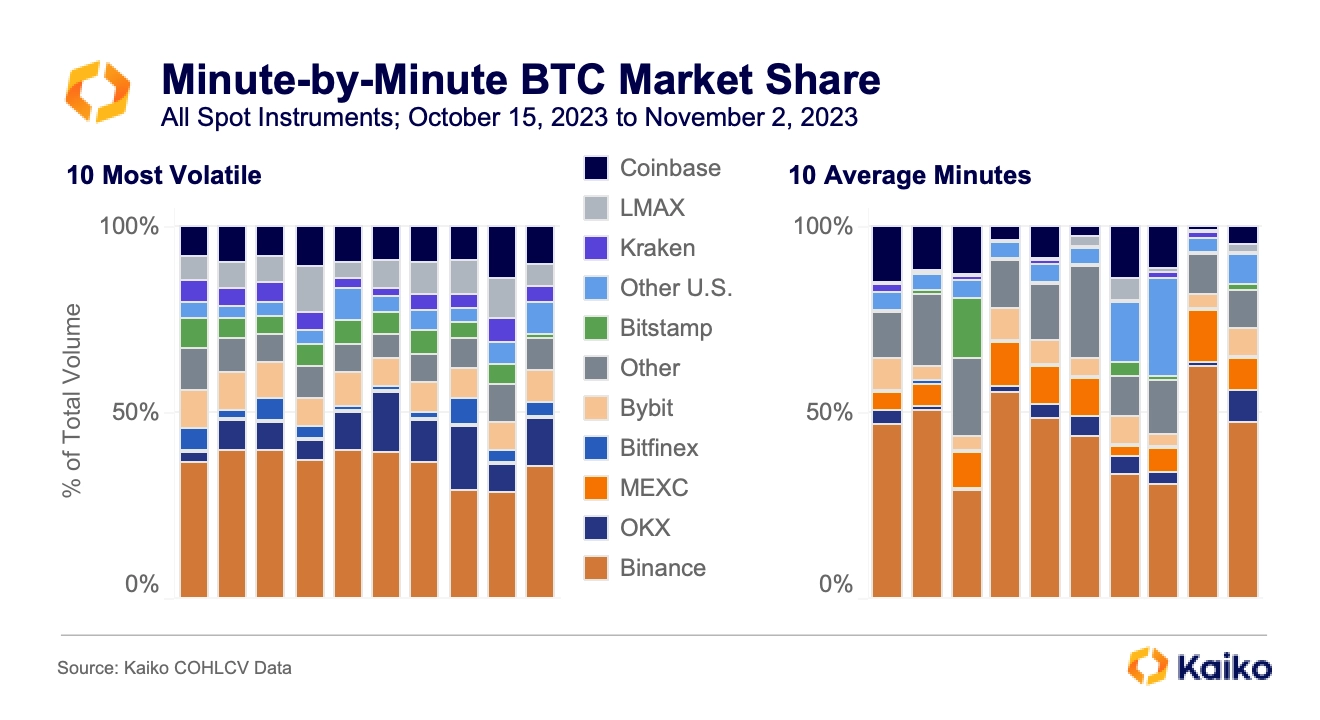
When evaluating these volumes, it's crucial to differentiate between superficially inflated trading numbers — often a result of wash trading or other manipulative practices — and genuine trading activity. This distinction helps in identifying exchanges that offer a realistic depiction of market dynamics and price discovery.
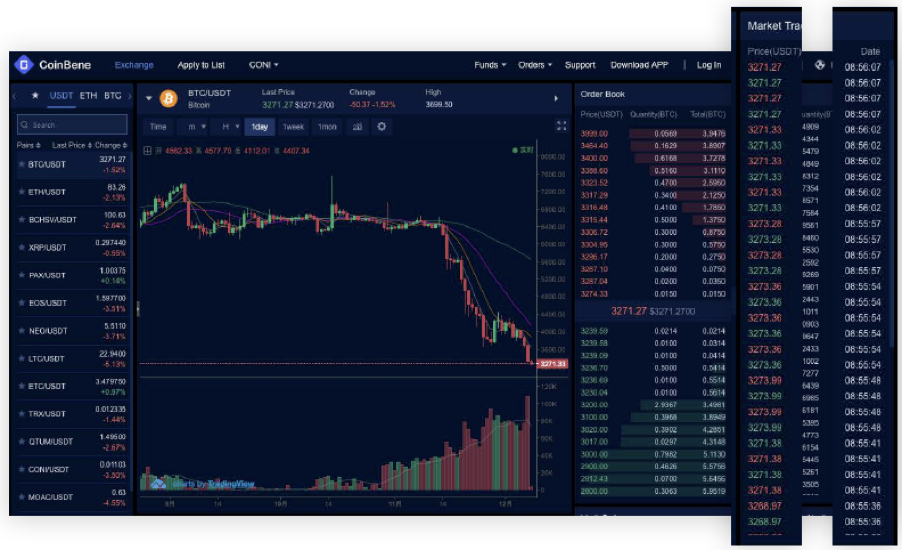
Moreover, exchanges characterized by consistently low depth are less desirable for inclusion in the Relevant Market. Market depth, measured by the volume of bids and asks at different price levels, is a critical component of market liquidity. Low depth indicates thin order books, where even small trades can cause significant price fluctuations, leading to higher volatility. Such conditions are not only detrimental to the stability and reliability of the market but can also mislead decision-making based on this skewed data.
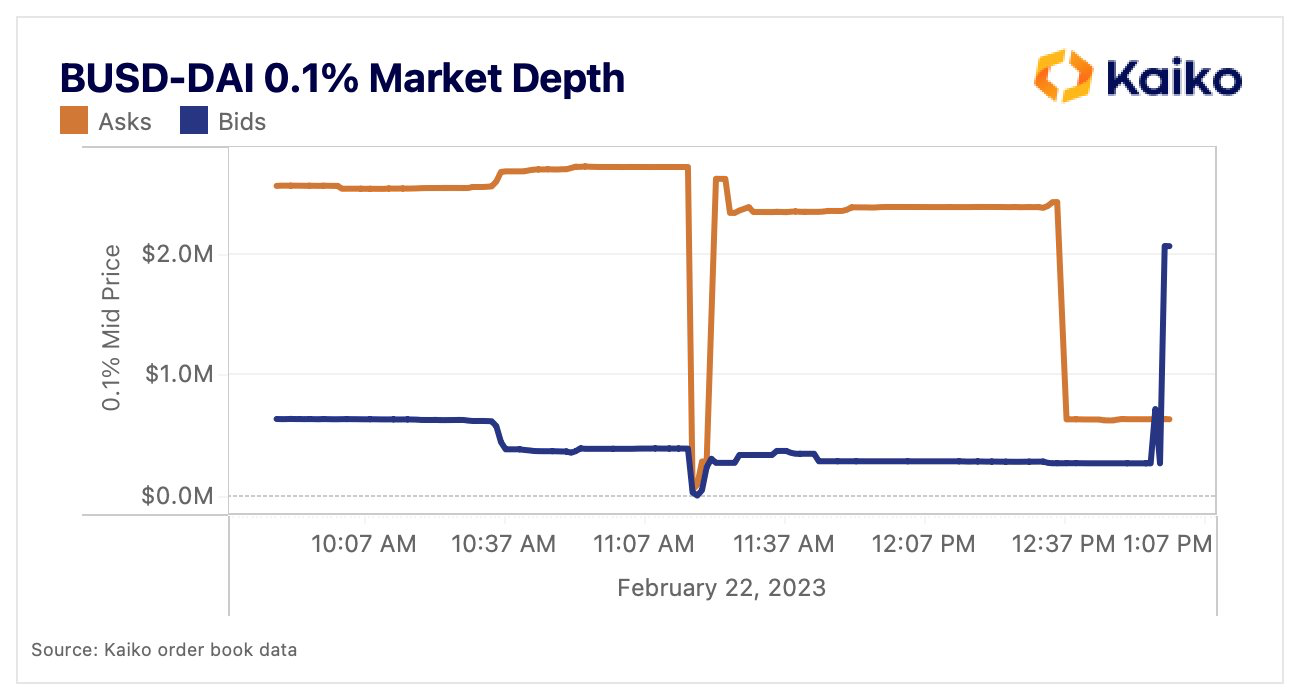
Meticulously filtering out both exchanges and instruments (a specific pair on a specific exchange, for example, BTC-USDT and BTC-TUSD on Binance are two separate instruments) with low depth enables great confidence in the ability to provide a resilient trading environment where prices reflect genuine market sentiments and are less susceptible to manipulation or sudden shifts due to limited order book depth. This rigorous selection process underpins the integrity of the Relevant Market, making it a true barometer of market conditions across the cryptocurrency landscape.
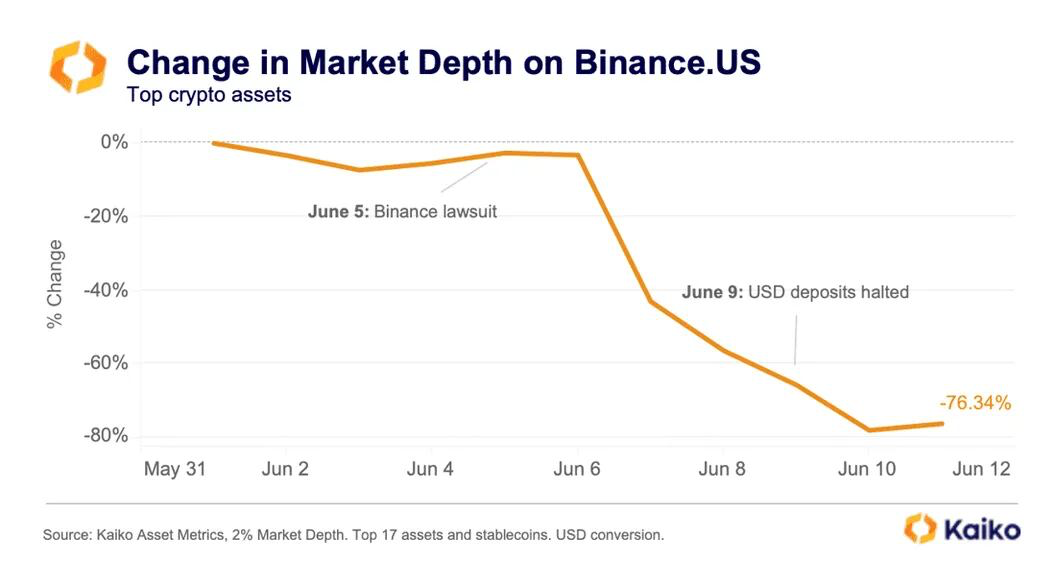
Access to and quality of all relevant market data
Access & Data Quality
Reliable access to both real-time and historical market data is an essential consideration for selecting exchange sources. High-quality data, with detailed granularity, allows for a precise analysis of market trends and behavior over different time frames. Furthermore, the availability of real-time order books with depth data, including Level 2 data provided through well-documented APIs, plays a critical role in assessing the immediate liquidity environment and market depth. This comprehensive data collection helps stakeholders verify the authenticity and accuracy of market information.
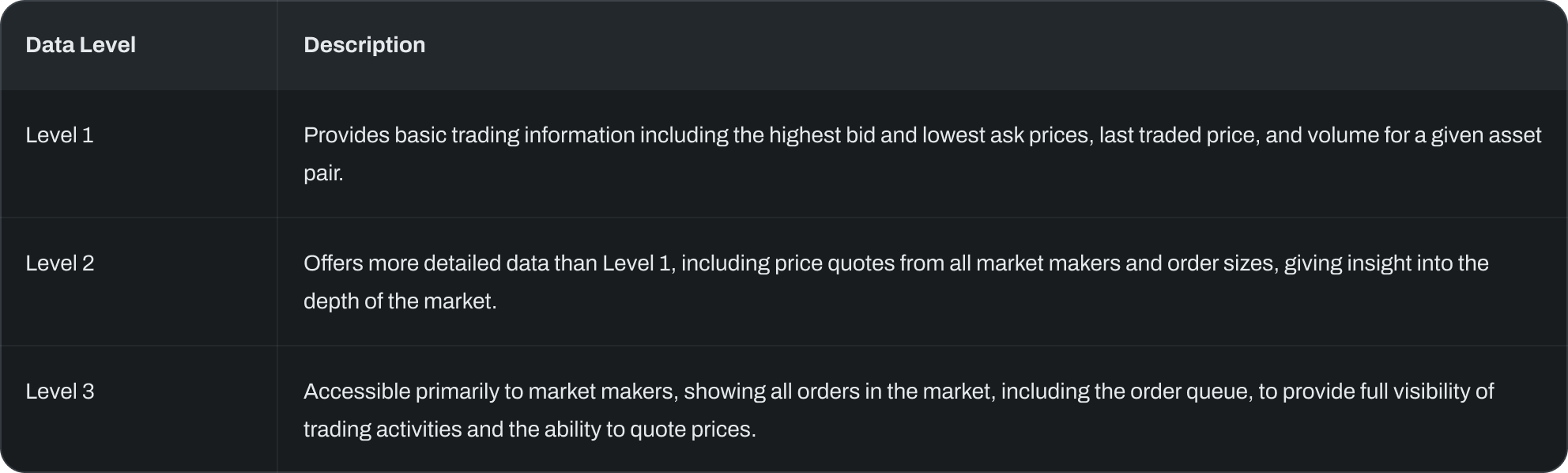
Liquidity & Market Quality
As discussed above, evaluating liquidity and market quality begins with a thorough analysis of exchange-level data, ensuring that less trustworthy exchanges are removed. Next, there must be a more granular analysis of instrument-level data. This includes monitoring the total volume over an extended time frame and ensuring that daily volumes consistently reach a minimum threshold that satisfies confidence in price stability (e.g. $3M average daily trading volume).
This stringent criteria ensures the framework focuses on exchanges and instruments with significant market activity, thereby minimizing the impact of smaller, potentially volatile trading platforms. To further refine this assessment, the framework employs a normalized trading volume metric that adjusts reported volumes based on web traffic analytics, which helps to identify suspicious trading. Statistical processes are also employed to detect unusual trading patterns. Additionally, market volume dispersion is analyzed using the coefficient of variation, confirming that 90% of daily volume fluctuations fall within two standard deviations, which serves as a reliable indicator of market stability.
The framework also meticulously evaluates key metrics specific to asset liquidity and market quality. This includes analyzing the spread at the top of the order book, which should remain competitive with other venues to ensure fair asset pricing reflective of the broader market. Slippage is another critical metric, measured by the simulated price movement created by orders of a significant size. Lastly, the framework assesses volatility by comparing the volatility profiles of markets like ETH/USD and ETH/BTC with those at reputable exchanges, ensuring that asset behavior aligns predictably across various market conditions.
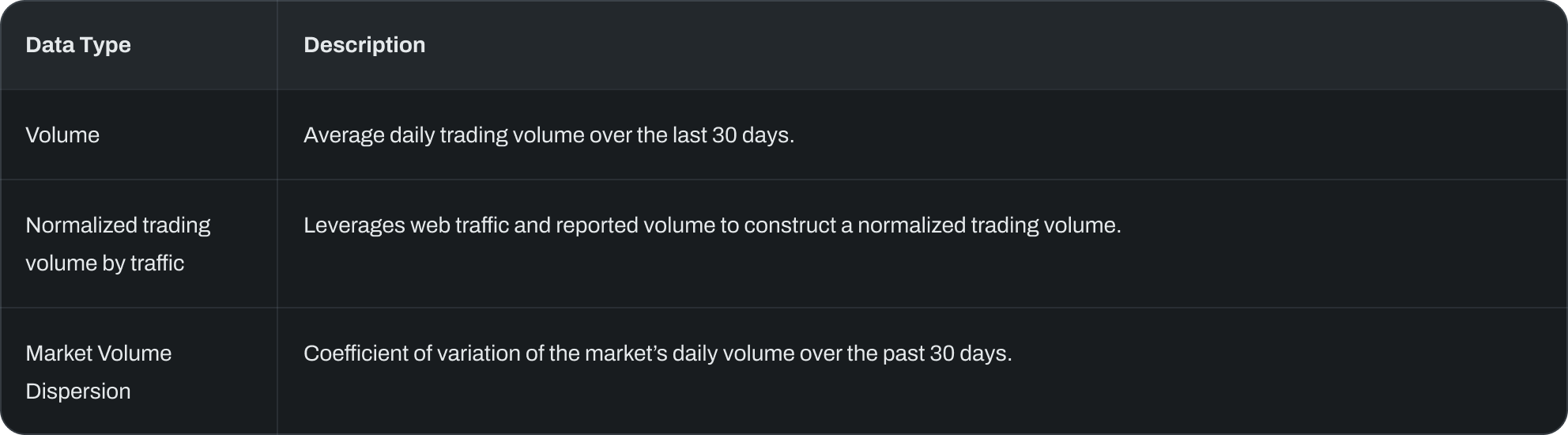
Technical and Security Robustness
In assessing technical issues that could influence the liquidity of relevant assets, it is critical to examine the technological infrastructure of exchanges. A key factor is the availability of data feeds through various channels like REST APIs, websocket feeds, or FIX protocols. Notably, the accessibility of websocket feeds enhances real-time data transmission, which is vital for maintaining liquidity during fast market movements. Another significant technical parameter is the incidence of downtime through API channels, with an ideal uptime rate of at least 99.99% being crucial to avoid disruptions in trading activities. Additionally, exchanges that maintain higher rate limits and shorter response times are better equipped to handle high-frequency trading environments, thus supporting more stable liquidity conditions.
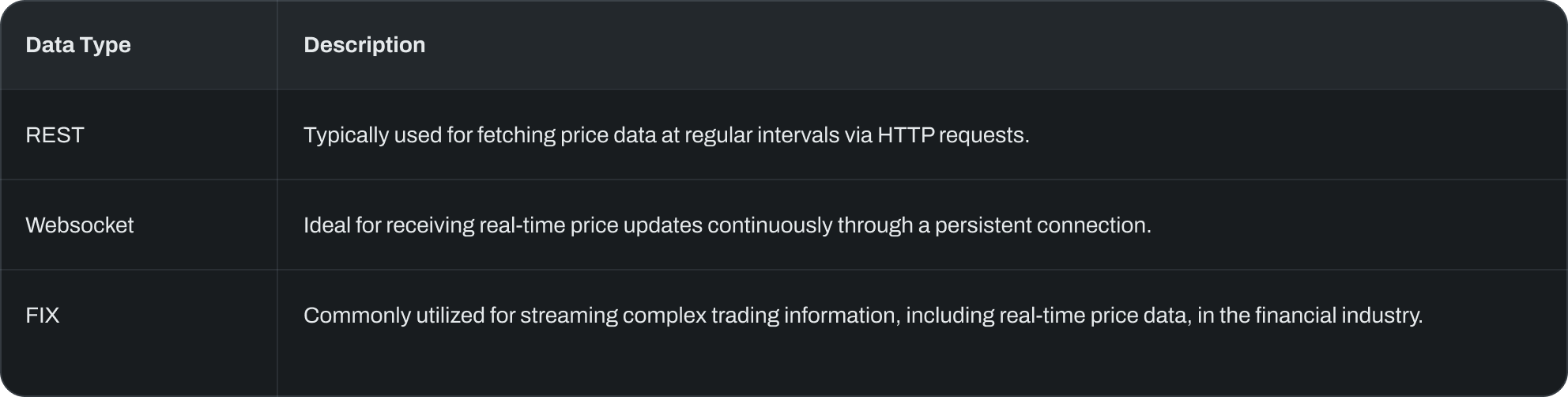
Security issues form a substantial part of the evaluation framework, given their potential to abruptly affect the liquidity of assets through breaches or operational disruptions. The adoption of stringent security certificates like SOC2 and ISO27001 is essential, as these standards signify robust data protection measures that an exchange has in place. Furthermore, the use of cold storage to safeguard assets offline considerably reduces the risk of theft and unauthorized access. User account protection mechanisms, such as two-factor authentication, advanced password policies, captcha, and phishing protections, are evaluated to determine the efficacy of an exchange in shielding its users from external threats. The framework also considers whether exchanges utilize a custody provider to secure crypto assets, enhancing overall security. Lastly, the historical frequency of suffered hacks, especially recent incidents within the last two years, plays a critical role in the assessment, with multiple or recent breaches leading to a lower score due to increased risk to asset liquidity and investor confidence.

Governance, Legal, Compliance
Governance, legal, and compliance factors are crucial in evaluating exchanges to ensure they operate within the bounds of legal and ethical standards, which ultimately impacts market trust and stability. Key considerations include the public availability of the legal exchange name in terms and conditions and on their website, which supports transparency and legal accountability. Moreover, the extent and detail of Know Your Customer (KYC) and Anti-Money Laundering (AML) procedures outlined in publicly accessible terms and conditions reflect the exchange's commitment to regulatory compliance and its ability to discriminate between different levels of KYC/AML guidance. The integrity of trading policies and the effectiveness of market surveillance activities, especially those managed by third-party entities, are also assessed to gauge how well market abuses are prevented and detected. The country in which an exchange is based is another important data point, determining which regulations the exchange is subject to and how these regulations are enforced; a centralized exchange should be registered as a regulated financial institution, money services business, or some other label with a trusted financial regulator. Finally, providing loss insurance through a third party or its own funds is used as an indicator of financial health and security.
Pricing Methodologies
This section critically examines traditional pricing methodologies such as Last Trade Price (LTP) and Volume Weighted Average Price (VWAP). It proposes alternative methods for achieving more accurate asset pricing and discusses the benefits of consolidating order books to establish benchmark pricing.
Last Trade Price
It may seem intuitive to use the Last Trade Price as a reliable indicator since it represents the most recently executed transaction. However, the Last Trade Price provides no guarantee that a user can replicate a trade for the same price. As a lagging indicator, Last Trade Price lacks real-time liquidity information, fails to accurately reflect the market structure, and often suffers from delays, all of which undermine its effectiveness in real-time pricing. Below a list of related incidents are examined.
Susceptibility to Flash Crashes
As any trader knows, flash crashes — and flash surges — can be unexpected and unforgiving. These are often caused by user error (”fat finger”), system error (an outage), low liquidity, or a combination of these factors. In the case below, INJ’s price on Kraken spiked 25% in a matter of seconds following a string of large market buys, which overwhelmed the order book. In this instance, Last Trade Price relying on Kraken alone failed to provide a complete view of the market, where prices were otherwise stable, leading to liquidations that otherwise would not have occurred.
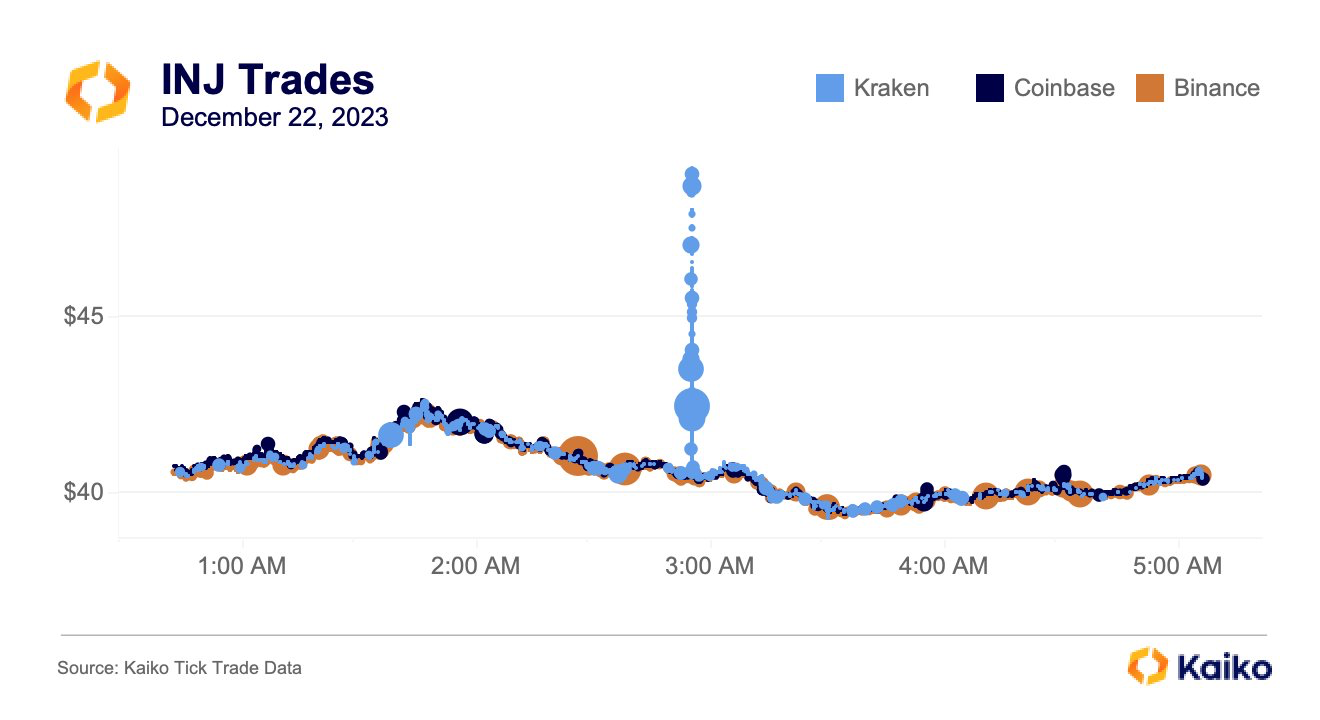
Susceptibility to Liquidations
Susceptibility to liquidations also poses significant risks. For instance, on September 13, 2022, a single exchange liquidation event on Kraken for ETH/USD cleared the order book on one side, causing the last trade price to drop from 1750 to 1630. Meanwhile, market-makers on other exchanges backstopped liquidity, and the best price available was only reduced from 1748 to 1670. Additionally, there have been incidents where institutional exchanges, relying on a single data provider, have erroneously liquidated their users due to a technical issue with the provider.
VWAP
Volume Weighted Average Price (VWAP) is often utilized as a pricing methodology on the premise that it provides a more comprehensive view of the market by averaging the price based on volume. However, similar to the Last Trade Price, VWAP comes with its own set of limitations that can affect its reliability as a robust price calculation method. Primarily, VWAP is a lagging indicator; it is computed using historical data, which may not accurately represent current market conditions. This delayed reflection does not capture the immediate liquidity or the ongoing structural changes in the market, thus potentially leading to discrepancies between the VWAP and the actual market price at which trades can be executed.
Moreover, the reliance on past transaction data means that VWAP can appear laggy even when applied over relatively short time frames. This lag can be particularly problematic in fast-moving markets, where prices can swing in seconds. Although VWAP is sometimes promoted for its resistance to manipulation compared to simpler metrics like Last Trade Price, it is still vulnerable, especially during periods of low liquidity. For example, in the AVAX/USD manipulation attack, the VWAP was manipulated by influencing the price through strategic, low-volume trades that disproportionately affected the average due to their timing during periods of low overall market activity.
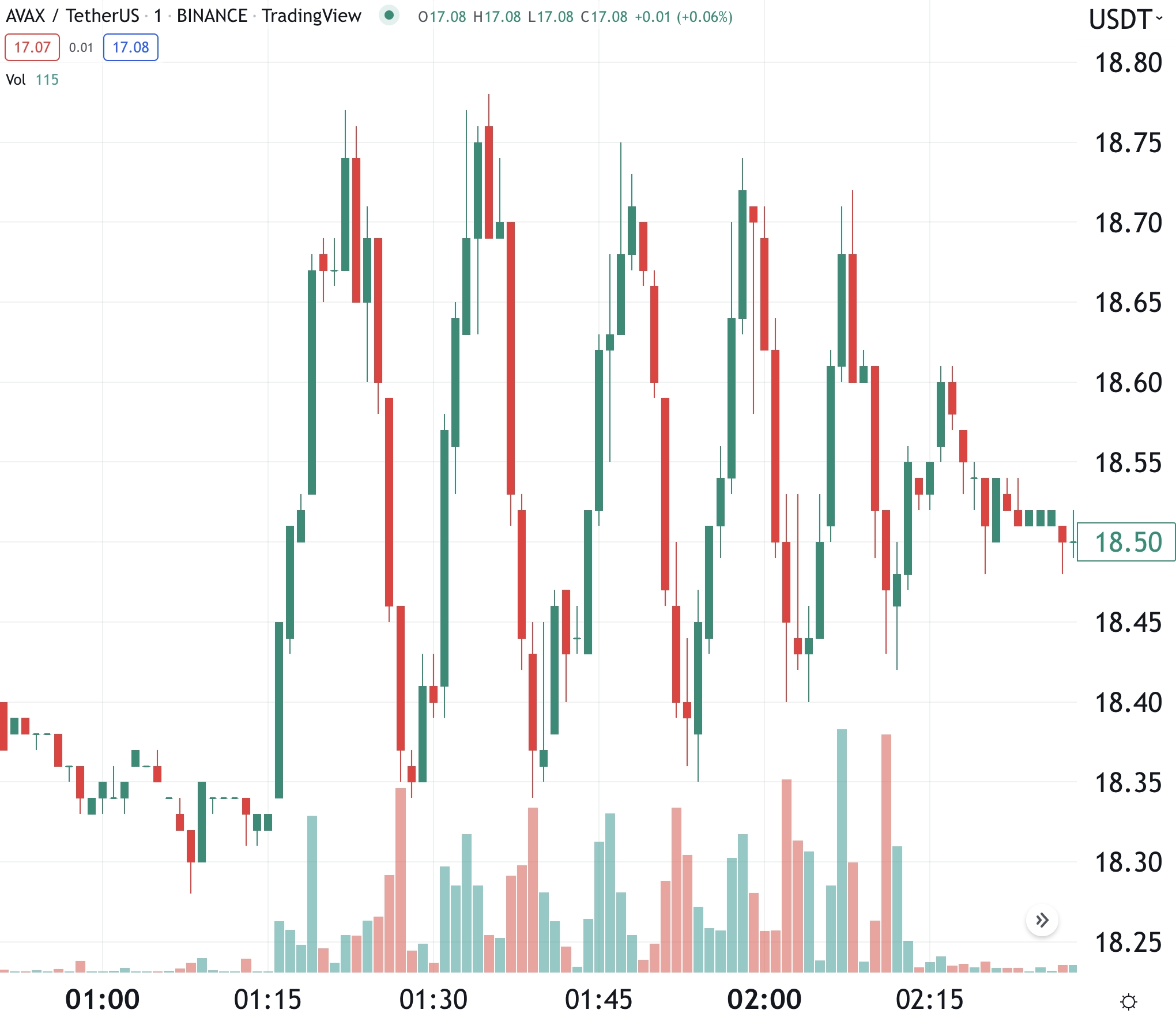
These characteristics highlight that while the VWAP methodology may offer some advantages in terms of integrating volume into price calculations, its effectiveness and reliability are significantly hampered by its inherent delays and susceptibility to market conditions. Therefore, relying solely on VWAP for pricing can lead to inaccurate valuations and misguided trading decisions, underscoring the need for more immediate and dynamic pricing methodologies in the evaluation of financial assets.
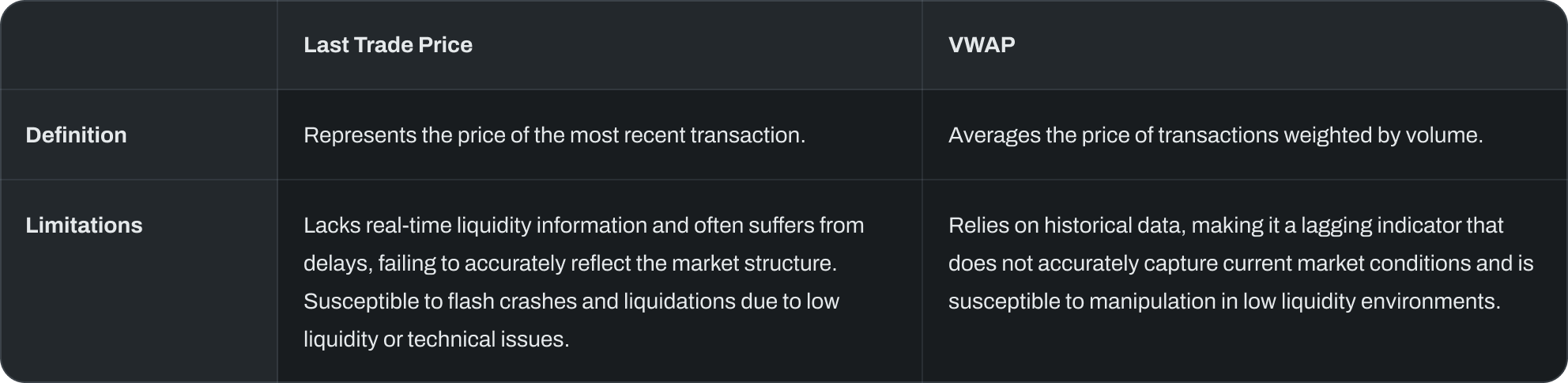
Alternative Pricing Methodologies
Liquidity-Weighted Pricing
In response to the limitations of traditional pricing models like Last Trade Price and VWAP, alternative methodologies that utilize instantaneous scans of market liquidity offer a more dynamic approach to price composition. These methods account for the immediate state of liquidity across various types of exchanges, whether centralized (CLOB) or decentralized (AMM/DEX). The key is to base the price calculations on the aggregate liquidity at the moment of calculation, reflecting the most achievable prices in the market.
In pricing methodologies, the volume-weighted and liquidity-weighted approaches represent two principal methods, each leveraging different aspects of market data to ascertain asset prices. The volume-weighted approach prioritizes prices at which higher volumes have been executed, focusing on the top of the order book and considering exchanges with substantial trading volumes as more reliable and less susceptible to price manipulation.
The liquidity-weighted approach examines the entire order book of constituent exchanges, emphasizing prices with substantial order quantities behind them. It focuses on mid-price orders to prevent manipulation and assigns weights based on the quantity available at each price level. This approach is forward-looking, reflecting insights into market liquidity and expectations about future market conditions.
While the volume-weighted approach offers a retrospective view based on completed transactions, the liquidity-weighted approach provides a real-time, predictive glimpse of market dynamics. This makes it particularly valuable for capturing the current state of market liquidity. These methodologies highlight the complexity of pricing in financial markets, where both past transactions and future expectations play critical roles in determining the most accurate prices.
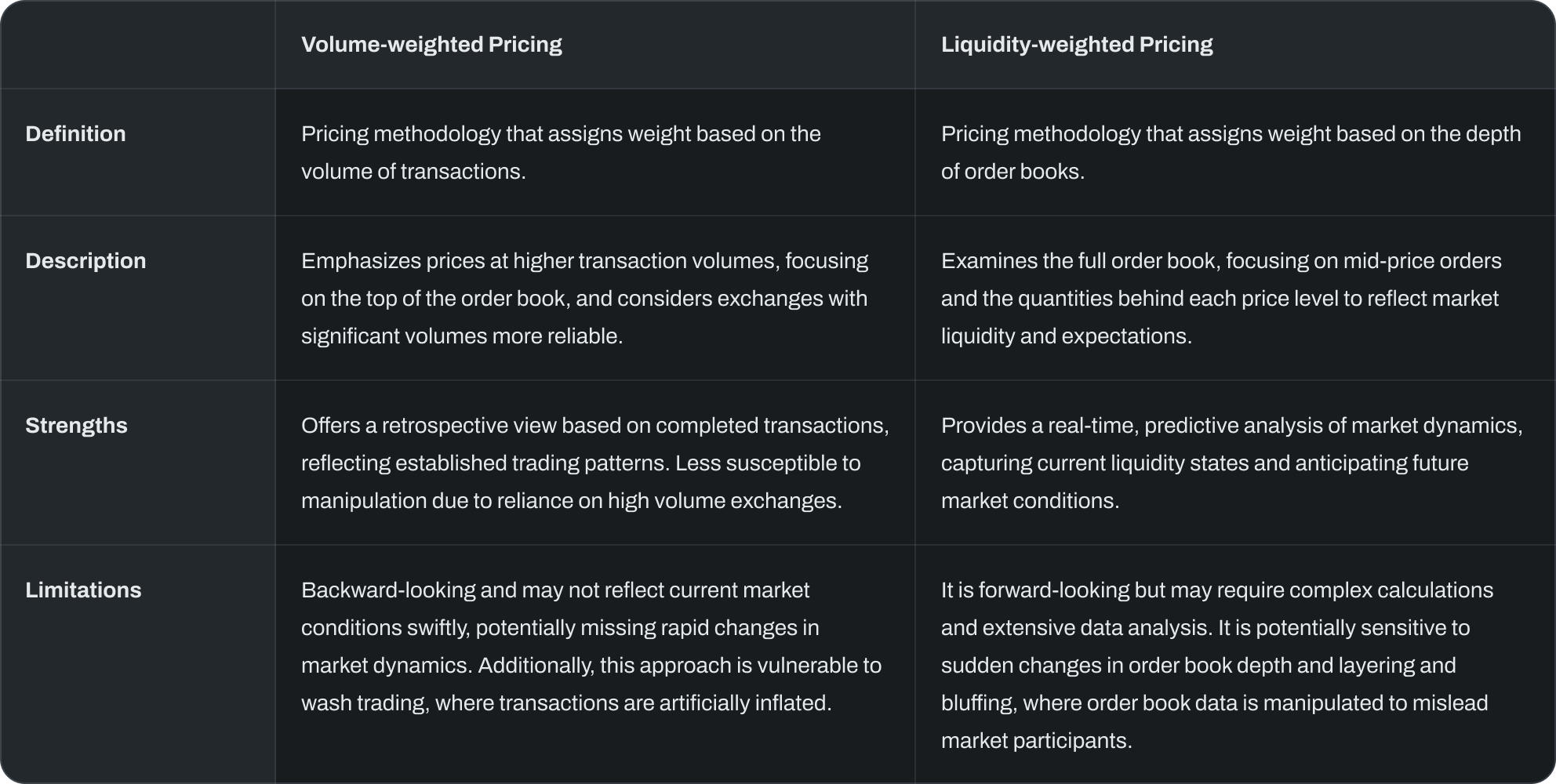
Price Aggregation Techniques
This section delves into advanced price aggregation techniques and their critical role in strengthening price composition methodologies. It outlines various approaches to price aggregation, offering concrete examples to illustrate how these techniques address the challenges posed by outliers and data anomalies. Additionally, this passage contrasts opinionated and unopinionated methods of price aggregation, highlighting the trade-offs involved in selecting either approach.
Case Study #1: Chronicle
Price Sourcing: Primary Sources
Chronicle only utilizes prices directly from exchanges to form the aggregate price. These sources are predetermined via token pair-specific data models, which the validators employ independently to retrieve the Last Trade Price (LTP), prior to aggregation. More specifically, these sources are premium exchanges that must pass ongoing checks on liquidity, volume, spread, and price deviation, such as Coinbase, Binance, Kraken, Gemini, Uniswap, Curve, and Balancer.
Aggregation Methodology: Median of Medians
Chronicle was the first to employ the median-of-medians aggregation methodology in 2017 and continues to use it today. Each Chronicle Validator takes a median of the LTP directly from the sources. They then sign and submit this price observation before the protocol generates a final aggregate price by taking the median of validator-submitted prices.
Chronicle take an unopinionated approach here, focusing on a combination of robustly designed data models and the median of medians to filter outliers. This approach guarantees simplicity and swiftness in price aggregation, absolving Chronicle of the responsibility to establish parameters that inherently reflect a subjective perspective on the typical range within which the price should reside. On the other hand, it means that the median price may be vulnerable when the data set includes extreme outliers, which can skew the median value. However, Chronicle aims to offset this risk through the design and maintenance of robust data models unique to each Oracle.
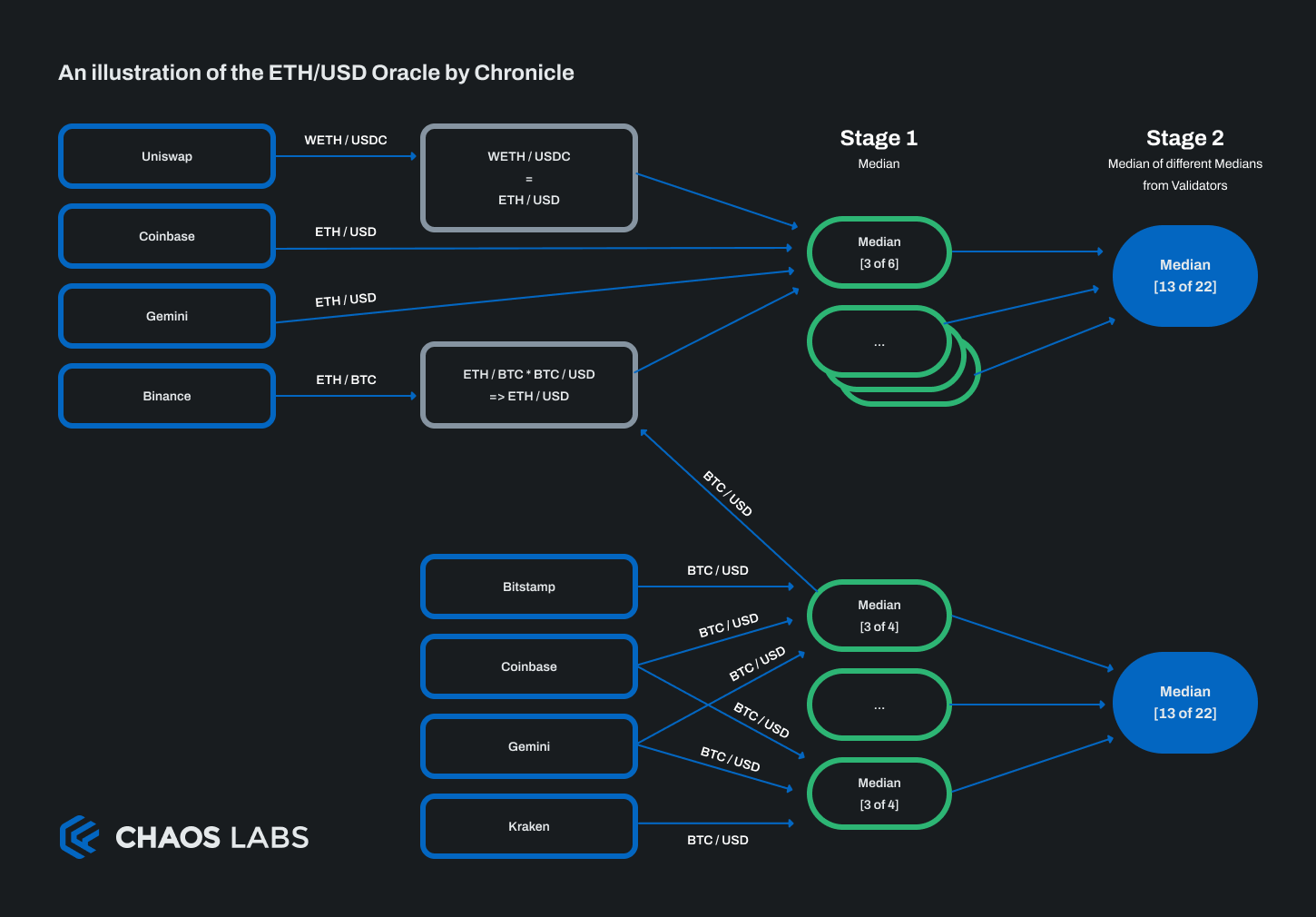
Averaging Methodology
Chronicle does not apply an averaging methodology. It prioritizes the dissemination of unaltered, high-fidelity prices and discloses the provenance of each aggregate price, which can be easily verified through its public-facing dashboard. This approach ensures Chronicle remains relatively unopinionated on the price, providing a direct portal into the most active public markets relevant to each supported asset.
Case Study #2: Chainlink
Price Sourcing: Secondary Sources
Chainlink Price Reports contain an aggregate price based on price data sourced from secondary sources. These sources are, more specifically, third-party premium data aggregators such as NCFX, Coin Metrics, Kaiko, and Tiingo.
Each aggregator has its own framework for deciding which exchanges to consider and its own proprietary statistical methods to identify suspect activity (e.g., order spoofing, wash trading, layering, outlier detection, and stale prices) and omit it from the final price calculation. Finally, each of these aggregators utilizes a VWAP or variation of a VWAP for consistency, to calculate a mid-market reference price.
Aggregation Methodology: Median of Medians
Chainlink's aggregation methodology produces the median of all participating nodes’ signed price observations. Each node’s observation represents a median price from n number of data sources. Chainlink seeks to be unopinionated here, focusing on the reliability of the aggregation process, outsourcing outlier detection to the data aggregators upstream. This method ensures neutrality and speed in price aggregation and means that Chainlink is not responsible for setting parameters that inherently project a subjective view of the normal bounds in which the price should sit.
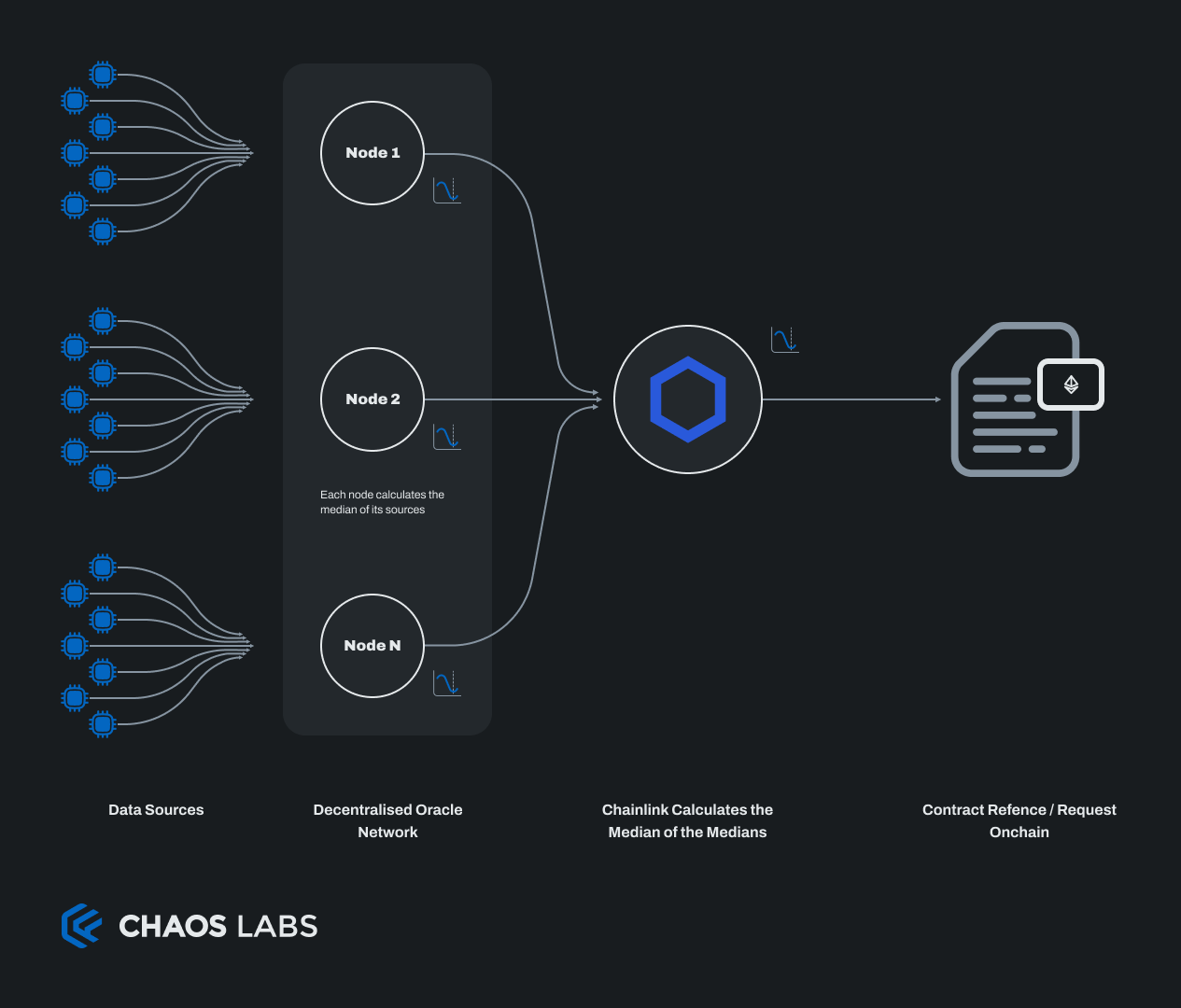
The median price by design excludes individual price outliers, but if there is sufficient disagreement in the market on what the current price is, the resulting aggregated median value will reflect that disagreement. An example of this would be the incident wherein the Chainlink wstETH/ETH market feed reported elevated price volatility resulting from a sandwich attack on Balancer v2. Some aggregators considered this volatility to be outside of the normal range and filtered out the trades. Others considered it meaningful trading activity on a primary constituent exchange of the Relevant Market and chose to include it. In cases such as this, Chainlink’s aggregation methodology ensures neutrality, rather than Chainlink taking an opinion on the price.
Averaging Methodology
Similar to Chronicle, Chainlink’s Off-Chain Reporting (OCR) protocol does not apply an averaging methodology to prices reported by oracle nodes. The Chainlink protocol remains unopinionated about the price, focusing on availability. The responsibility of price creation is outsourced to data sources upstream.
Case Study #3: Pyth
Price Sourcing: Primary Sources
The Pyth Network sources its data directly from primary data sources, such as exchanges and market makers including CBOE, Binance, Jump Trading, and Jane Street, as well as the data aggregators mentioned above. Each of these publishers submits a price and confidence interval.
Aggregation Methodology: Weighted Median
In contrast to Chronicle and Chainlink, Pyth takes an opinionated approach. The Pyth Oracle Program employs an algorithm designed to aggregate price feeds, with the aim of achieving robustness against manipulation, accurate weighting of data sources, and reflecting price variation across different venues in the aggregate confidence interval.
The algorithm calculates the aggregate price by giving each publisher three votes: one at their stated price and one each at their price plus and minus their confidence interval. The median of these votes is used to determine the aggregate price. Additionally, the aggregate confidence interval is calculated by selecting the larger distance between the aggregate price and the 25th or 75th percentiles of the votes. This approach uses the median to mitigate the impact of outliers and adjusts the confidence interval based on the precision of different data sources.
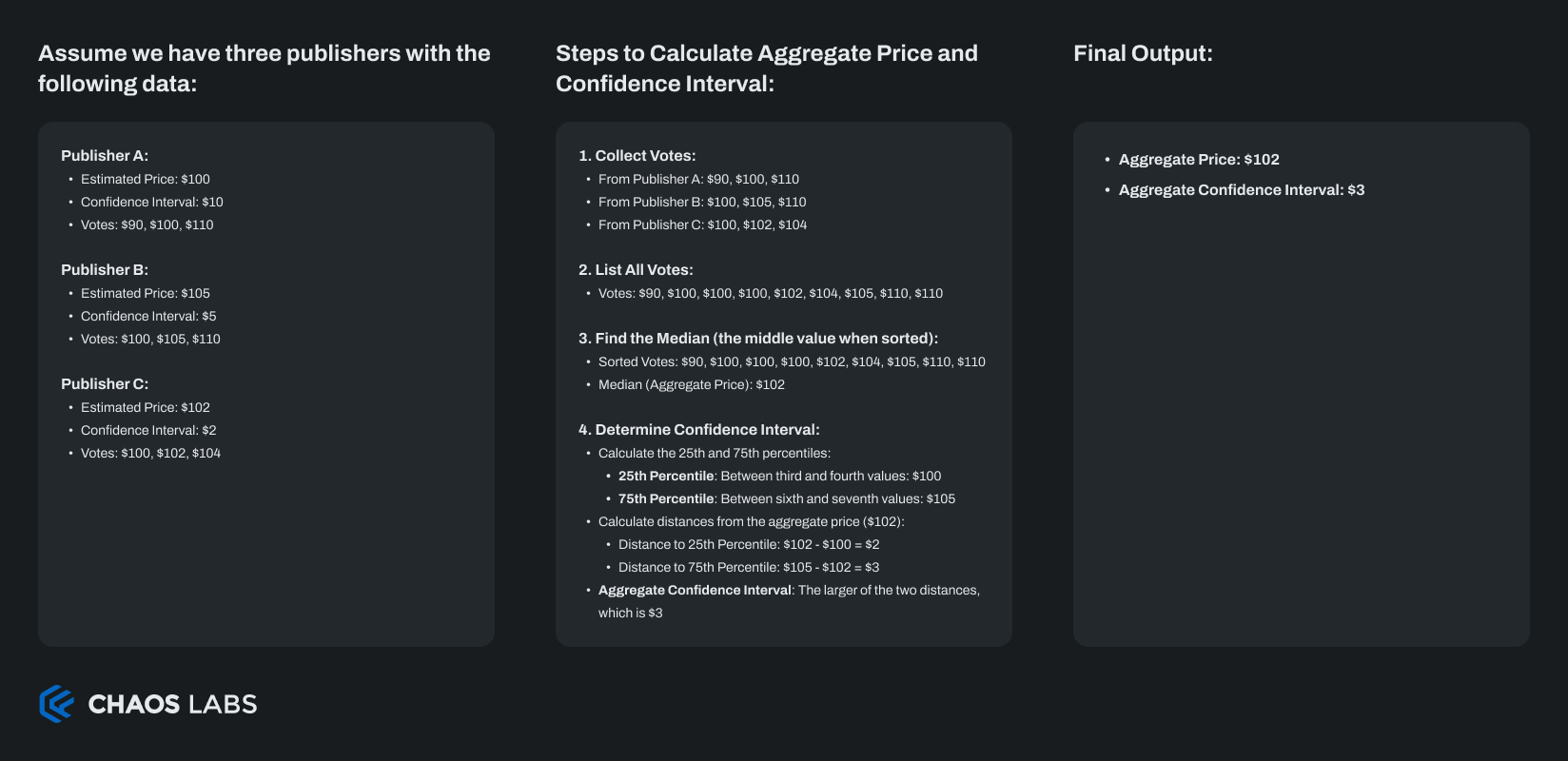
The methodology aims to produce consistent results across various scenarios and is based on theoretical properties that adapt the traditional median calculation to include confidence intervals. This technique may be useful in deriving a more stable and reliable median, reducing the chances of significant deviations caused by aberrant data points. An example of the limitation of this approach is shown in the diagram below, where the lack of consensus among data points highlights the challenges faced even with sophisticated filtering methods.
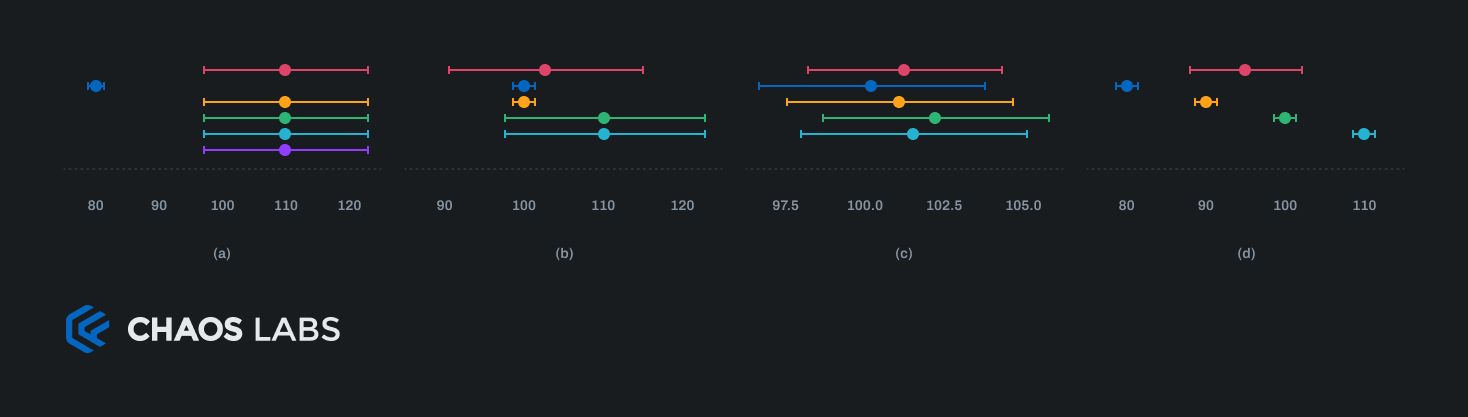
Averaging Methodology: Adapted EMA
Pyth utilizes an adapted Exponential Moving Average (EMA) approach to average price data, designed for on-chain applications where computational efficiency is crucial. This method modifies the traditional EMA by incorporating a slot-weighted, inverse confidence-weighted system, which assigns lesser weight to price samples with wider confidence intervals, effectively minimizing their impact on the overall average. This slot-based timing mechanism aligns with the Solana Virtual Machine’s (SVM) operational framework, using specific slot counts to approximate time periods. The averaging methodology also considers the potential correlation of errors across different slots to ensure a more conservative estimation of the confidence interval, which reflects the reliability of the EMA. This approach aims to maintain stability and accuracy in the averaged price data without over-emphasizing outliers.
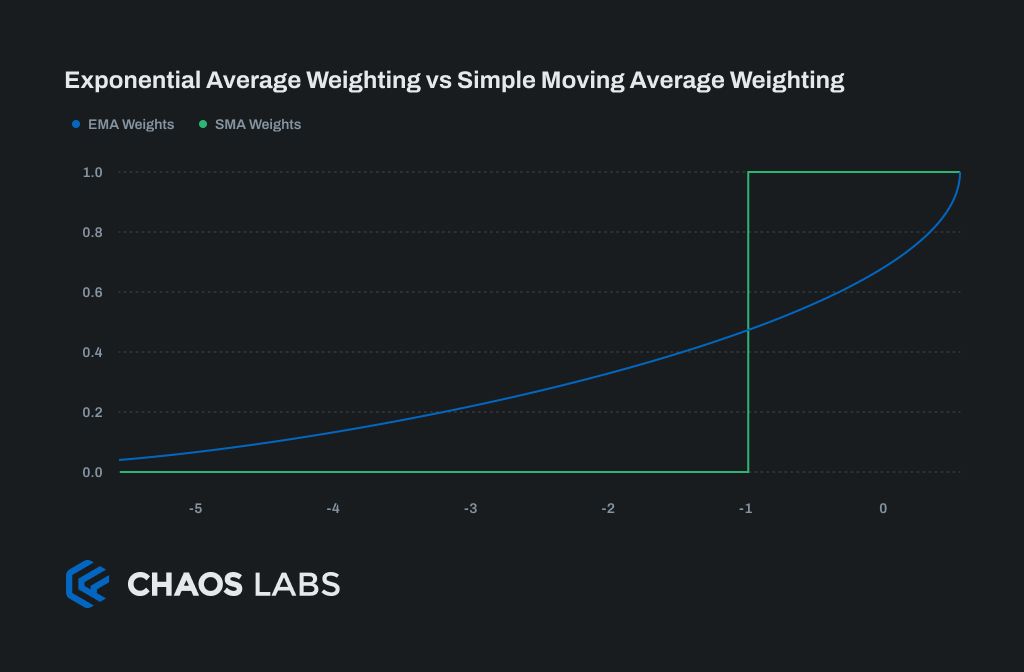
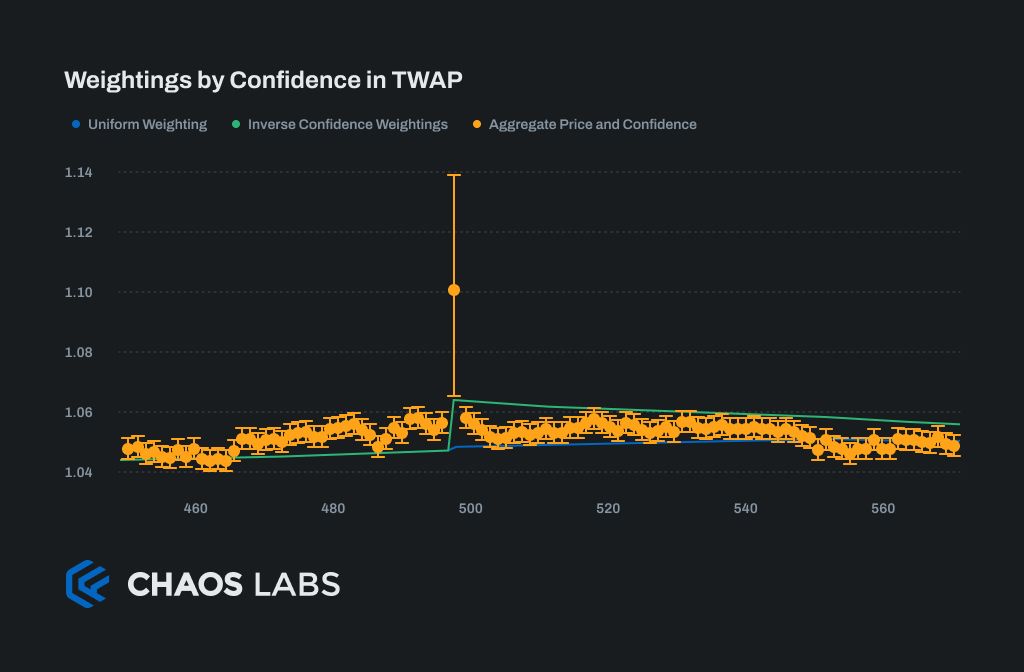
Taking an opinionated view on the price presents a set of tradeoffs. While advanced price aggregation techniques can result in a more robust and outlier-resistant output, the presence of any issues or bugs in this aggregation process is inherited by consuming applications downstream.
The potential consequences of this tradeoff have been highlighted a couple of times in the case of Pyth; once when a bug in the underlying floating point math library used by Pyth’s on-chain program to compute the TWAP, resulted in an overflow that led to inaccurate TWAP calculations and another time when BTC-USD flash crashed 90%, due to “two different Pyth publishers publishing a near-zero price and the aggregation logic overweighting these publishers’ contributions,” as outlined in the Pyth Root Cause Analysis.
Case Study #4: RedStone
Price Sourcing: Primary & Secondary Sources
RedStone derives its prices from a combination of primary and secondary data sources, using proprietary infrastructure called “fetchers” to retrieve prices directly from DEXes, CEXes and data aggregators.
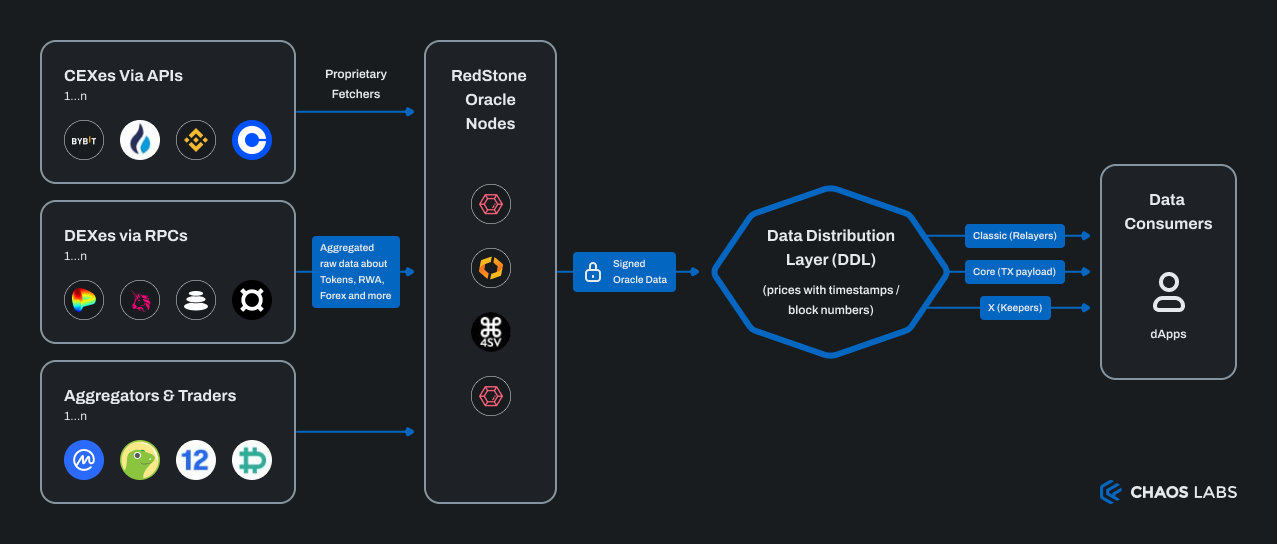
Aggregation Methodology: Sanitized Median
RedStone implements a two-stage data aggregation process, split into an off-chain and on-chain workstream:
- Off-chain: Each RedStone Oracle Node must prepare and publish a manifesto that describes which sources are used and what the aggregation parameters are. The data is first sanitised based on basic correctness requirements (non-zero numeric values) and more advanced constraints which differ per asset (e.g. bid/ask spread or DEX slippage). This activity is followed by data filtering where clear outliers that significantly differ from others or past values are removed.
- On-chain: Data from multiple RedStone Oracle Nodes is then aggregated in the second phase, which occurs on-chain in the data feed smart contract. In most cases, the values are medianized; however, consuming protocols are free to overwrite this basic aggregation strategy.
Averaging Methodology
RedStone currently supports a few different averaging methodologies, including a TWAP and market-depth-adjusted price, however, these must be manually selected by consuming protocols. The default option is the sanitized median.
Price Source or Messenger?
The key difference between opinionated and unopinionated oracles is in how they handle and process data, which in turn influences whether they act more as a "price source" or a "price messenger." Opinionated oracles, such as Pyth and RedStone, actively determine the bounds within which they believe the price should fall. They employ algorithms at the aggregation layer to detect and remove data they deem as outliers, using subjective parameters to mould the final price. This approach positions them as a price source—they don't just relay data, they shape it.
In contrast, unopinionated oracles like Chainlink and Chronicle defer to the upstream data providers for rigorous data sanitization and outlier detection. They do not alter the data but reflect it as it comes from the sources, aiming to mirror the market consensus without imposing their own view on what the price should be. This makes them more of a price messenger, delivering data with minimal interference, which is valuable for protocols that prefer a pure reflection of market conditions.
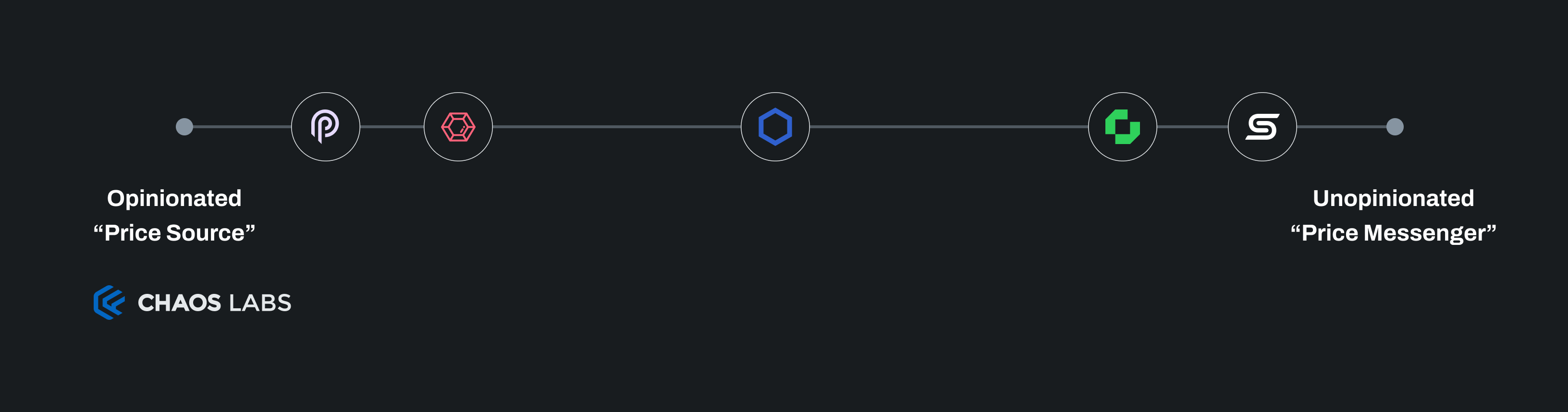
The choice between using an opinionated or an unopinionated oracle depends on a protocol’s specific needs. Protocols that perform their own data verification processes may opt for unopinionated oracles. Conversely, protocols that prefer to receive pre-processed and adjusted data might choose opinionated oracles, which filter and tailor the data according to predefined criteria.
Unfortunately, not all applications are aware of these distinctions, which can lead to mismatches between their operational requirements and the oracle services they use. Educating protocol developers on these differences is crucial to ensuring they make informed choices about oracle providers that impact their applications' functionality and trustworthiness.
Addressing Illiquid Assets
Addressing quality issues at the source is crucial for illiquid assets. Precise data inputs are vital—consider, for instance, constructing prices that accurately reflect the implications of buying or selling $10,000 of a token. Without this attention to detail, no aggregation method can adequately compensate for fundamentally flawed or inaccurate data. An illustration of this issue can be seen with the MNGO asset, where the poor quality of data significantly impacted the reliability of aggregated prices.
The role of oracles in asset pricing also introduces complexities. Oracles may create a false sense of security by suggesting robust pricing for instruments that are fundamentally illiquid. This situation leads to tension between protocols seeking an oracle price feed for a new asset and oracle providers grappling with the decision to assume the responsibility of pricing a highly volatile and illiquid asset. A critical factor in this equation is the oracle's stance: a more opinionated oracle takes on greater responsibility and faces increased scrutiny for reporting prices that include trading activities or price movements considered outliers by market observers.
Stablecoins, LSTs and LRTs
Assets such as LSTs and, more recently LRTs, which offer a yield-bearing equivalent while representing a correlated underlying asset, often encounter controversy regarding their pricing logic at the protocol level. These assets are anticipated to closely track or precisely match a peg, and they are typically priced under fixed price assumptions to mitigate short-term volatility. The underlying assumption is that these assets will swiftly revert to their mean, enhancing capital efficiency and reducing liquidation risks.
However, there's a counterpoint to consider. These assets can potentially deviate from their correlated underlying for extended periods, or even indefinitely. This divergence can occur during periods of long withdrawal queues, continuous slashing events, exploits, or when the underlying stablecoin's economics suggest a departure from the peg. Thus, an alternative approach is to use a market-priced oracle, which can accurately determine the asset's value. This setup enables liquidation events in case of a swift depeg, aligning with the current market price. However, since oracle providers aggregate market prices, especially when assets such as LSTs and LRTs face high amounts of relative leverage, this aggregation can intensify price drops. Consequently, users may face a subpar experience, and there's a heightened risk of accumulating bad debt, as seen in the recent ezETH depeg incident. Stablecoins, however, often don't fit into this category due to the generally limited relative leverage demand.
Hence, the decision regarding the pricing logic of these assets should be embedded within the respective protocol itself. This decision should account for the protocol's debt internalization capabilities and parameterization, alongside considering the collateral demand and utilization on lending platforms as well as the specific debt assets involved. Additionally, external mechanisms may be employed to further mitigate risks surrounding tail events, such as enforcing bounds wherein new LST/LRT positions cannot be opened if a depeg exists, as well as capping the underlying price of a stablecoin to mitigate exclusively price manipulative outcomes.
Conclusion
Chapter 3 extensively reviews key tenets of price composition methodologies and the implications different design decisions have for Oracle Risk and Security. It emphasizes the criticality of a robust exchange evaluation framework, highlighting how substantial trading volume and stringent compliance standards are indicative of reliable exchanges that enhance pricing accuracy. Discussing various pricing methodologies like LTP, VWAP, and liquidity-weighted pricing, the chapter reveals their strengths in real-time market reflection and vulnerabilities like susceptibility to rapid market changes. It differentiates between opinionated and unopinionated oracles, illustrating how their data processing influences their functionality as either price influencers or passive messengers, impacting oracle reliability. Moreover, it addresses the complexities of pricing illiquid assets, stressing the precision needed in data collection to ensure the stability and security of these price compositions.